Comprehensive Guide to Auto Tagging Software
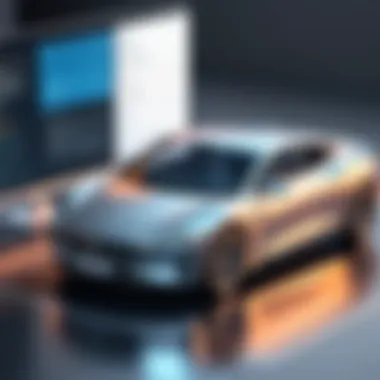
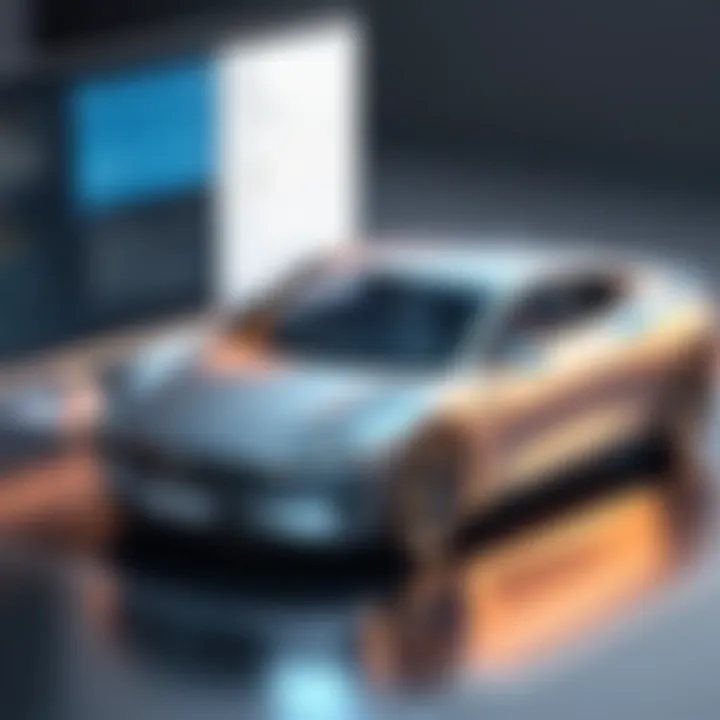
Intro
In today's digital age, managing large volumes of data efficiently is crucial for organizations. Auto tagging software has emerged as a vital tool in this respect. It automates the process of tagging and categorizing data, enabling easier organization and retrieval. This guide will explore various aspects of auto tagging software, emphasizing its functionalities, implications for productivity and organization, and methods for selecting the right tool for specific needs.
Functionality
Overview of key features
Auto tagging software possesses several key features that enhance its efficiency. These include:
- Automated tagging: The software can automatically assign tags to content based on predefined rules or learned patterns.
- Machine learning capabilities: Many platforms utilize machine learning algorithms to improve tagging accuracy over time.
- Bulk tagging: Users can often tag multiple items simultaneously, saving valuable time.
- Integration options: Software that seamlessly integrates with existing systems can simplify workflows.
The effectiveness of auto tagging software is determined largely by its features. High-quality software will also provide user-friendly interfaces, allowing business professionals to navigate easily without excessive training.
How well the software meets user needs
Businesses vary widely in terms of their needs and expectations from auto tagging software. Organizations focused on marketing may benefit from software that emphasizes social media integration and engagement metrics. On the other hand, data-driven companies might prioritize accuracy and speed in large datasets.
Auto tagging software must be optimized for user needs. Features such as customizable tagging rules and flexible categorization can cater to different sectors effectively. By focusing on user experience, developers can enhance the overall effectiveness of the software.
Scalability
Adaptability for growth
Auto tagging solutions must be adaptable to meet the changing needs of businesses as they grow. A scalable software will accommodate increased data volume without compromising performance. Companies should consider solutions that can adjust to fluctuations in data needs.
Options for additional features or modules
Choosing auto tagging software with modular capabilities can significantly enhance value. As businesses evolve, the ability to add new features or modules is essential. Modules for specific functions, such as analytics or reporting, can provide deeper insights and foster better decision-making.
Understanding Auto Tagging Software
Auto tagging software represents a critical tool in the realm of data management and digital organization. It automates the process of assigning tags to files, images, and other digital assets, which facilitates easier categorization and retrieval. This technology is not merely a convenience; it significantly influences productivity, lending organizations a competitive edge by enhancing their data organization methods.
Definition and Purpose
Auto tagging software refers to applications specifically designed to automatically generate identifying tags for various forms of content. By utilizing algorithms and artificial intelligence, these tools analyze data attributes and context to apply relevant tags. The primary purpose of such software is to streamline the categorization process, reducing the manual effort required to manage large amounts of data. The implementation of auto tagging leads to improved workflows for teams handling vast digital resources. This in turn enhances overall productivity and allows users to focus on strategic tasks that require human judgement.
Historical Context
The evolution of auto tagging software can be traced back to the rise of digital media and the need for effective management systems. In its early stages, tagging was predominantly manual, requiring significant human input which was both time-consuming and error-prone. With advancements in machine learning and natural language processing, the concept of auto tagging emerged. Companies recognized that automating this process could help in managing the burgeoning volumes of digital content. Over the years, the technology has matured, with many solutions now integrated into content management systems, digital asset management platforms, and e-commerce sites. This history highlights a shift from manual categorization to a more efficient automated process, reflecting broader trends in digital innovation.
Mechanics of Auto Tagging
The mechanics of auto tagging are essential to understanding how this software operates and its significance in various industries. Auto tagging systems utilize a combination of algorithms, machine learning, and natural language processing to automatically assign relevant tags to digital content. These tags help in categorizing and organizing content, enhancing its discoverability. Understanding the mechanisms behind auto tagging can provide insights into its effectiveness and applicability in real-world scenarios.
How Auto Tagging Works
At its core, auto tagging software functions by analyzing the content it processes and identifying key themes, topics, or attributes. This is typically achieved through several steps:
- Content Analysis: The software examines raw data, be it text, images, or videos. It focuses on identifying patterns and keywords that signify the main subjects.
- Tag Generation: Using predefined rules and machine learning models, the software generates appropriate tags based on the analysis. These tags may relate to the content type, context, or other metadata.
- Learning from Input: Many auto tagging solutions incorporate feedback mechanisms, where the system learns and adapts from user input. This continuous learning process ensures that the tagging becomes more accurate over time.
- Implementation: Once tagging is completed, the software integrates tags into the content management systems, making the content readily accessible to users.
Understanding these steps is crucial for businesses considering implementing auto tagging. Improved accuracy in tagging translates to better organization and retrieval of digital assets.
Technology Behind Auto Tagging
The technology that powers auto tagging software involves various sophisticated components:
- Natural Language Processing (NLP): This technology allows the software to understand human language, facilitating the extraction of meaningful information from text. NLP helps in discerning context, sentiment, and intent, which are critical for accurate tagging.
- Machine Learning Algorithms: These algorithms learn from vast datasets to identify what tags have been effective in the past, refining the tagging process over time.
- Image Recognition Software: For multimedia content, image recognition tools analyze visual elements, recognizing objects, text, or patterns, thus enabling tagging based on visual cues.
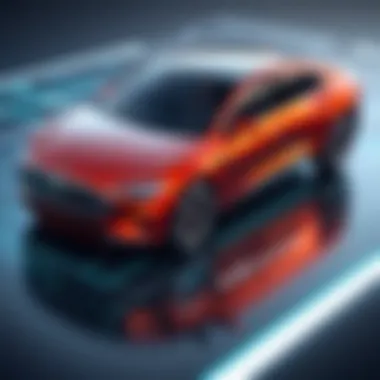
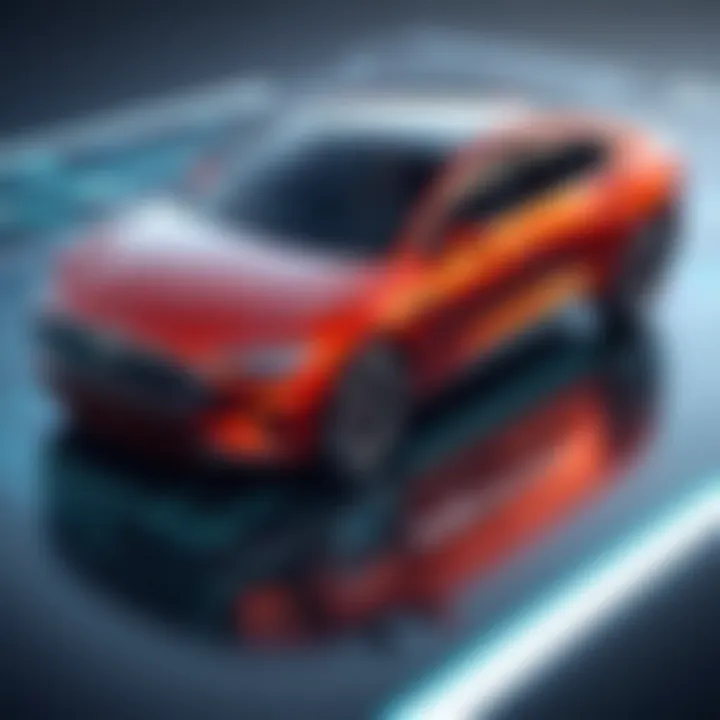
The integration of these technologies enhances the software's capabilities, enabling it to tag content with high precision. As a result, organizations can benefit from improved content management workflows and more relevant content discovery experiences.
"Auto tagging not only saves time but can also reduce errors that arise from manual tagging, ensuring a higher level of consistency across tagged assets."
In essence, understanding the mechanics behind auto tagging sheds light on its value in contemporary digital landscapes.
Benefits of Implementing Auto Tagging Software
Auto tagging software provides numerous advantages for businesses seeking to streamline operations and enhance data management. As organizations grapple with increasing volumes of data, implementing effective solutions becomes crucial. Auto tagging helps automate tedious tagging processes, thus allowing companies to focus on their core activities. Below, we explore key benefits that arise from the adoption of auto tagging software.
Efficiency Through Automation
One primary advantage of auto tagging software is the significant improvement in efficiency. Manual tagging is labor-intensive and time-consuming. By utilizing automated solutions, organizations can reduce the time spent on data organization and tagging tasks. This increase in operational efficiency leads to faster decision-making and enables teams to allocate resources more effectively.
Moreover, automation helps eliminate human error, which can occur during manual tagging. Consistency in tagging is essential for accurate data categorization. With auto tagging, the software maintains a uniform approach to tagging, resulting in a more reliable data set. This, in turn, supports better reporting and analytics capabilities.
Improving Data Organization
Data organization is vital in today's information-driven world. Auto tagging software enhances how organizations manage and categorize their data. By assigning tags automatically based on defined criteria, the software ensures that information is systematically organized. This streamlined approach minimizes the chaos that often accompanies manual tagging methods.
Additionally, improved data organization facilitates easier access to relevant information. Users can quickly locate specific data types, thus reducing time spent searching through large datasets. As a result, employees can devote more time to valuable tasks that drive business objectives forward.
Enhancing Searchability
The power of searchability cannot be overstated in any operational framework. Auto tagging software enhances the searchability of digital assets across platforms. With accurate tagging, users can easily find the information or resources they need without navigating complex file structures.
When tags are applied consistently and correctly, it improves overall discoverability. In the context of mixed or extensive content libraries, this enhanced searchability is a game changer. Users can execute targeted searches based on keywords or categories, leading to higher productivity and faster retrieval of information. Ultimately, this capability fosters a more informed and responsive business environment.
"Automating the tagging process elevates how teams can work with data, providing clarity and focus on strategic goals rather than repetitive tasks."
Use Cases for Auto Tagging Software
The utility of auto tagging software extends across various domains, each benefiting from enhanced organization, productivity, and efficiency. Understanding the specific use cases for this technology is critical for businesses aiming to optimize their workflows and enhance data management. By leveraging these software solutions, organizations can streamline processes, improve information retrieval, and ultimately drive growth. Below, we explore three prominent areas where auto tagging software plays an essential role.
Digital Asset Management
In the realm of digital asset management (DAM), auto tagging software significantly simplifies the process of organizing large volumes of files. With digital assets ranging from images to videos and documents, manual tagging can be a daunting task. Auto tagging automates this process by analyzing the content and assigning appropriate tags based on established criteria. This not only saves valuable time but also ensures consistency in tagging across all assets.
For example, platforms like Adobe Experience Manager utilize auto tagging to facilitate better content discovery and retrieval. The software identifies key elements within assets, helping users find specific items quickly. Furthermore, this streamlined tagging mechanism supports better collaboration among creative teams, making it easier to manage and share resources effectively.
Content Management Systems
Content management systems (CMS) also significantly benefit from auto tagging software. As organizations generate vast amounts of content, managing this information efficiently becomes increasingly important. By using auto tagging, content creators can automatically categorize articles, blog posts, and other forms of content based on predefined keywords and themes.
This not only enhances searchability within the CMS but also improves user experience. For instance, WordPress users can implement plugins that leverage machine learning algorithms to generate tags, leading to improved content organization. Consequently, the overall structure of the website becomes more intuitive, making it easier for visitors to navigate through relevant content without unnecessary delays.
E-commerce Platforms
In e-commerce, the demand for effective categorization of products is paramount. Auto tagging software assists platforms like Shopify and Magento in sorting products into various categories and subcategories, ensuring that consumers can find what they seek swiftly. By automatically generating tags based on product descriptions and attributes, businesses save time and reduce the chances of human error.
Additionally, effective tagging enhances the customer experience. With better organization, shoppers can filter and search for products more effectively, improving conversion rates. Data gathered from auto tagging can also be analyzed to gain insights into shopping patterns, allowing businesses to tailor their offerings and marketing strategies accordingly.
Efficient auto tagging can result in a smoother shopping experience, leading to increased customer satisfaction and retention.
In summary, auto tagging software is increasingly integral to functions within digital asset management, content management systems, and e-commerce platforms. By understanding and implementing the use cases of this technology, businesses can improve efficiency, organization, and the overall user experience.
Evaluating Auto Tagging Solutions
Evaluating auto tagging solutions is a critical step for businesses looking to implement this technology effectively. The choice of software can significantly influence the success of business processes, from content organization to data retrieval. This section focuses on essential elements to consider when evaluating such solutions. Businesses must analyze features, capabilities, and overall value to ensure a right fit with their operational needs.
Key Features to Consider
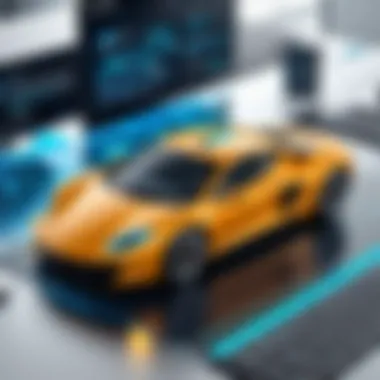
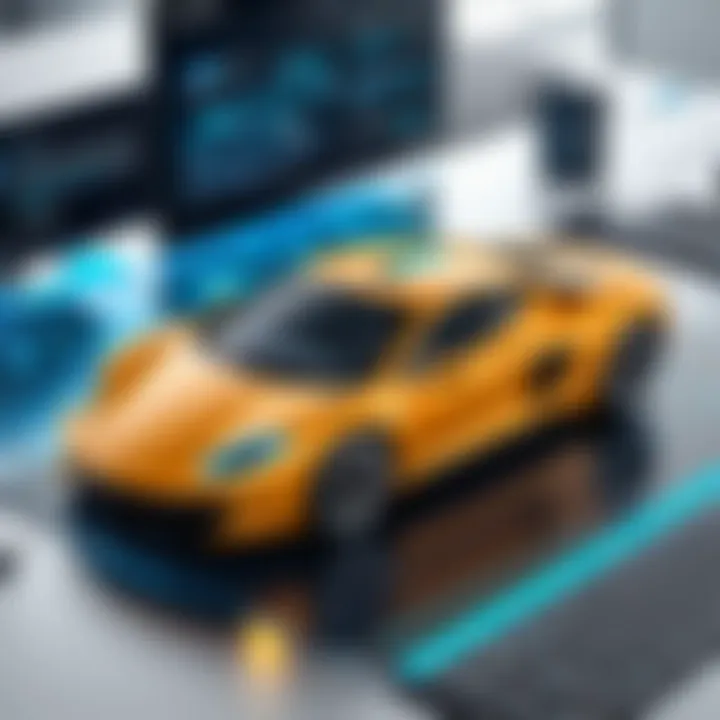
When exploring auto tagging software, certain key features should be prioritized:
- Accuracy: The software must provide high precision in assigning relevant tags. Automated tagging systems should minimize erroneous tags that could lead to confusion.
- Scalability: As organizational needs grow, the software must be capable of handling increased data volumes without performance degradation.
- Integration Capabilities: The ability to work seamlessly with existing systems, like content management systems or digital asset management platforms, is vital.
- User Interface: An intuitive user interface can enhance user adoption and reduce training costs.
- Real-time Processing: For many applications, the ability to tag data in real-time can significantly improve workflow efficiency.
- Customization Options: Businesses might require specific tagging criteria suitable for their unique content or data types.
These features play a fundamental role in determining the appropriateness of the software for specific business landscapes.
Comparative Analysis
After identifying potential auto tagging solutions, a comparative analysis should be conducted. This analysis serves to weigh the pros and cons of each solution against one another. Consider the following methods for effective comparison:
- Feature Comparison: Create a comparison chart to visualize the capabilities of different software options. This should include the aforementioned key features such as accuracy and integration capabilities.
- User Feedback: Investigate user reviews and testimonials. Platforms like Reddit and industry forums can provide insights into user experiences.
- Cost-Benefit Analysis: Evaluate the software pricing against its features and capabilities. This analysis helps to determine the return on investment a particular software solution may offer.
- Trial Versions: Many software providers offer trial versions. Testing these versions allows organizations to assess usability and suitability before making a financial commitment.
By systematically evaluating these elements, businesses can make informed decisions that align with their specific requirements and strategic goals.
"Making an informed choice regarding auto tagging software is crucial for optimizing business efficiency and data management."
Integrating auto tagging solutions into existing operations can lead to greater productivity and improved organization. Businesses must, however, take the necessary steps to evaluate their needs accurately to select the most suitable solution.
Challenges Associated with Auto Tagging
Despite the numerous advantages that auto tagging software offers, it is important not to overlook the challenges associated with its implementation. These challenges can impact both the effectiveness of the software and its overall contribution to organizational processes. Understanding these obstacles is essential for businesses that wish to optimize their use of auto tagging solutions. Key challenges often include issues related to accuracy and relevance, as well as integration with existing systems.
Accuracy and Relevance
One of the most pressing concerns with auto tagging software is accuracy. The software must be able to analyze content effectively to produce tags that are relevant and meaningful. If the tags generated are inaccurate, it can lead to confusion and inefficiencies.
- Inaccurate Tags: Inconsistencies may arise when the software misinterprets the content, resulting in tags that do not reflect the actual context or meaning. This misalignment can hinder searchability and make it difficult for users to locate relevant information.
- Relevance Over Time: The relevance of certain tags can change over time. Trends and terminology evolve, and software that does not adapt to these shifts can become ineffective. Regular updates and adjustments to the tagging algorithms may be necessary to ensure ongoing relevance.
Organizations need to be aware that a reliance on auto tagging is not a substitute for human oversight. Regular checks and balances can assure that the tags are both accurate and relevant, maintaining the integrity of the entire system.
"Automation in tagging is powerful, but accuracy must remain a priority, or the organization may face significant challenges in data discovery."
Integration Issues
Successful implementation of auto tagging software requires seamless integration with existing systems and tools. However, integration poses its own set of challenges, which can complicate the user experience and limit the software’s effectiveness.
- Compatibility with Legacy Systems: Many organizations still operate using legacy systems that may not be fully compatible with modern auto tagging solutions. This can create hurdles in data transfer and functionality. If the software cannot effectively communicate with these existing systems, it can lead to fragmentation and data silos.
- Training and Adoption: Integrating new software typically requires training personnel to effectively use the tagging system. If users experience difficulty or resistance to change, the benefits of automation may not be fully realized. Lack of adoption can result in underutilization of the software, thereby leading to wasted resources.
- Ongoing Maintenance: Monitoring and maintaining the integration points are critical as updates occur. As software and systems evolve, maintaining compatibility and functionality can become labor-intensive.
In summary, while the adoption of auto tagging software can enhance productivity and data organization, organizations must be prepared to navigate the complexities of accuracy and integration challenges. By acknowledging these potential pitfalls, businesses can implement more effective strategies that align with their broader goals.
Future of Auto Tagging Technology
The rapidly evolving landscape of auto tagging technology has significant implications for various industries and for business efficiency. Understanding the future of this technology is crucial, especially in environments where data management is becoming increasingly complex. As organizations generate vast amounts of digital assets, auto tagging solutions are essential for maintaining order and enhancing productivity.
Emerging Trends
Recent advancements indicate several important trends shaping the future of auto tagging.
- Machine Learning and AI Integration: Machine learning algorithms are becoming integral in enhancing the accuracy of tag suggestions. As these algorithms learn from user behavior and tag application, they refine their suggestions over time, making the process smarter and more tailored.
- Natural Language Processing: The integration of natural language processing (NLP) is transforming how auto tagging software interprets and categorizes content. This technology allows the software to understand context and semantics, leading to more relevant tags based on content nuances.
- Real-Time Tagging Capabilities: Businesses increasingly require instant tagging as content is generated. Future auto tagging solutions will likely focus on real-time processing to ensure that tagging occurs as quickly as content gets created or modified.
- Personalization Features: As user experience becomes paramount, expect to see features that allow users to customize tagging preferences. Tailoring tags according to individual or departmental needs can significantly enhance usability.
- Multi-Platform Integration: Seamless integration with various platforms is another trend. Future software must connect easily with existing systems like CRM tools, social media platforms, and cloud storage services, making data management simpler and more effective.
Predictions for Development
As we look to the future, it is essential to identify where auto tagging technology is heading.
- Increased Focus on Data Privacy: With growing concerns surrounding data security and privacy, future auto tagging software will prioritize compliance with regulations such as GDPR. Enhanced data protection features will be vital to ensure user trust.
- Improved User Interfaces: User interfaces will see innovation focused on usability. As technology continues to advance, software designed for diverse user levels needs to be intuitive without sacrificing functionality.
- Expansion of Use Cases: More industries will realize the benefits of auto tagging, leading to an expansion in use cases. From healthcare to entertainment, unique applications will emerge that showcase the versatility of tagging solutions in managing varied digital assets.
- Collaboration Features: As teams become more global and dispersed, collaboration features will be crucial in auto tagging software. Future products will enable team members to tag and categorize collaboratively, enhancing efficiency globally.
- Sustainability Considerations: As businesses shift focus towards sustainability, there will be a trend toward eco-friendly software practices. Auto tagging solutions may incorporate features promoting green practices in data handling and management.
The evolution of auto tagging technology bears critical importance for businesses as they seek to streamline operations and improve data management efficiency.
As a conclusion, the future of auto tagging technology is bright, with a plethora of innovations anticipated. Businesses that stay ahead of these trends can significantly enhance their productivity and data management capabilities.
Case Studies of Successful Implementations
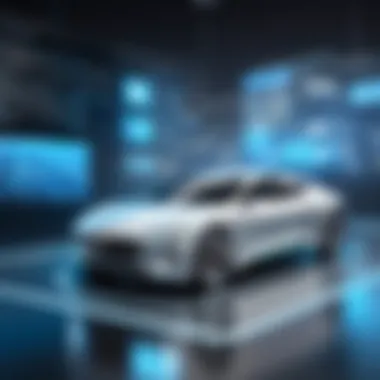
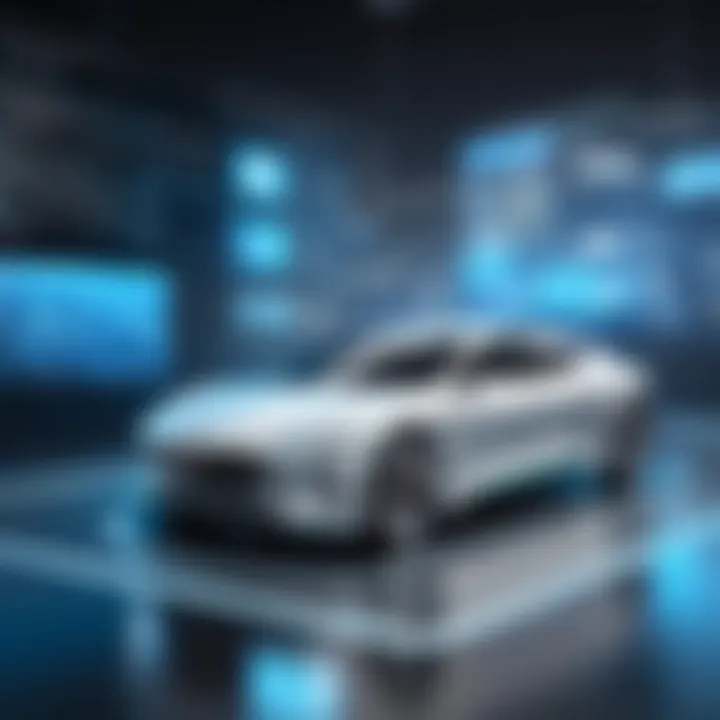
In the realm of auto tagging software, case studies present a pivotal resource for understanding practical applications and tangible outcomes. They reflect the real-world experiences of organizations that have adopted such technology. This section aims to showcase various industry-specific applications and user testimonials that underline the effectiveness and benefits of utilizing auto tagging software.
Industry-Specific Examples
- Media and Entertainment: The media industry often deals with vast catalogs of content. A notable example is Getty Images, a global visual media company. They implemented auto tagging software to manage their extensive library of photographs and videos. By automating the tagging process, Getty Images improved the speed of asset retrieval by over 30%, allowing clients to find relevant content efficiently, thus enhancing overall satisfaction.
- E-commerce: In the e-commerce sector, Shopify has leveraged auto tagging for product inventory management. By using intelligent algorithms for automatic tagging, Shopify allows merchants to organize products based on attributes like size, color, and season. This strategy not only optimizes the user experience but also leads to significant increases in sales conversion rates, demonstrating the software's value in driving business outcomes.
- Finance: Goldman Sachs adopted auto tagging for managing large volumes of financial documents. With software capable of automatically tagging reports to match regulatory requirements, they enhanced compliance and reduced the risk of errors. This case highlights the dual benefits of increased operational efficiency and adherence to complex regulations.
User Testimonials
Feedback from users of auto tagging software can provide invaluable insights. Testimonials reveal how these tools have transformed their organizational processes.
"Using auto tagging software from Cloudinary has revolutionized how we deal with media assets. The time saved on manual tagging allows our team to focus on creative tasks, leading to better productivity."
— Maria Johnson, Digital Marketing Manager at a leading advertising firm.
Tom Hart, the IT director at a comprehensive digital library, notes, "Our integration of Amazon Rekognition has automated our tagging process. We have reduced the time spent on image categorization by 70%, allowing curators to spend more time on content quality rather than data management."
These examples and testimonials illustrate various benefits of auto tagging software across multiple industries. Clearly, organizations that embrace these technologies often amplify their efficiency, enhance compliance, and optimize user experiences.
Integration with Existing Systems
Integration with existing systems is a crucial aspect of auto tagging software. In today’s fast-paced digital environment, businesses seek solutions that seamlessly fit within their operational framework. This integration ensures that organizations can maximize the utility of auto tagging while minimizing disruptions in their workflows.
The interface between auto tagging software and current systems influences the efficiency with which data is managed. Compatibility with other tools enhances the overall functionality of auto tagging solutions, enabling a more streamlined operation. Here are some specific elements to consider when evaluating integration:
- Compatibility with Current Tools: The software should work well with existing platforms such as content management systems, digital asset management tools, and e-commerce platforms.
- Ease of API Usage: A robust API allows for easier and more effective communication between different software solutions. This is essential for facilitating a smooth transfer of information, thus maintaining data integrity across platforms.
- Scalability: As businesses grow, their needs evolve. Auto tagging solutions need to accommodate scaling without requiring a complete overhaul of the current system.
- User Training and Support: Comprehensive support ensures staff members can easily adapt to the new software, enhancing overall productivity.
Benefit of such integrations include reduced manual labor, consistent data application, and improved search capabilities. The bottom line is that efficient integration leads to better user experience and maximizes the return on investment for software implementations.
APIs and Third-Party Tools
Using APIs and third-party tools is integral to enhancing the effectiveness of auto tagging software. APIs serve as bridges that connect different systems, allowing them to communicate effectively. For starters, they enable automatic data exchange, reducing the need for manual input. This not only saves time but also decreases the probability of error.
Many popular software solutions come equipped with APIs designed specifically for integration. For example, platforms like Salesforce or WordPress offer APIs that facilitate smooth interactions with auto tagging software. This can lead to significant improvements in workflow efficiency.
In addition, leveraging third-party tools can complement the core auto tagging functionality. Tools like Zapier can automate repetitive tasks by linking various applications together. When utilizing these tools, companies should ensure they have clear guidelines for data governance and privacy considerations to maintain compliance with regulations.
Maximizing Interoperability
Maximizing interoperability is vital in ensuring that auto tagging software works seamlessly within an organization's tech ecosystem. Interoperability refers to the capability of different software systems to communicate and exchange information effectively, thus enhancing overall efficiency.
To achieve better interoperability, organizations should focus on the following aspects:
- Standardized Data Formats: Utilizing common data formats simplifies the exchange of information between applications. JSON and XML are often used to maintain consistency.
- Real-time Syncing: Solutions that offer real-time updating capabilities can significantly reduce delays in data accessibility. This is beneficial for time-sensitive operations.
- Comprehensive Documentation: Having well-documented processes and integration points aids IT teams as they implement and maintain auto tagging solutions.
"Successful integration transforms disparate systems into a unified interface, enriching user experience and productivity across the board."
Investing in interoperability not only alleviates integration headaches but also leads to a more agile and responsive organization. When software can interact fluidly, businesses are better positioned to adapt to changing market demands.
Regulatory Considerations
In an era where data management is paramount, understanding regulatory considerations for auto tagging software is crucial. Organizations increasingly rely on automated systems for handling vast amounts of data. However, with automation comes the responsibility of ensuring compliance with regulations, which can differ significantly by region and industry. Addressing regulatory frameworks can enhance user trust and prevent potential legal issues.
It is essential for businesses to recognize the guidelines set forth by entities like the General Data Protection Regulation (GDPR) in Europe and the California Consumer Privacy Act (CCPA) in the United States. These regulations dictate how data can be collected, used, and managed. Non-compliance may result in significant penalties, thereby emphasizing the need for regulatory awareness in auto tagging software solutions.
"The lack of adherence to regulatory considerations can not only harm a company’s reputation but also lead to severe financial repercussions."
The integration of regulatory considerations into the software development life cycle fosters an environment of proactive compliance. Ensuring that auto tagging software aligns with applicable laws reassures stakeholders that the business takes data governance seriously.
Compliance Requirements
Achieving compliance with relevant regulations involves implementing specific practices in the auto tagging process. Organizations must incorporate features that ensure accountability and transparency. For instance, auto tagging software should include mechanisms that allow users to trace how data was tagged and processed.
- Documentation: Maintaining thorough records of data processing activities is essential. This documentation should detail how tagging decisions are made, which can aid in audits and regulatory reviews.
- User Consent: Obtaining explicit consent from users for data processing ensures compliance with regulations such as GDPR. Auto tagging systems should have clear pathways for users to give or revoke consent easily.
- Data Minimization: Only the necessary data should be collected and tagged. This is a critical principle under several regulations, which helps limit the risk of data breaches.
- Regular Audits: Conducting periodic assessments to ensure that the auto tagging processes adhere to compliance mandates can help identify areas that require improvement.
Data Privacy Concerns
Data privacy is a pivotal consideration when deploying auto tagging software. The handling of personal and sensitive information necessitates robust privacy measures. Organizations must implement controls that support the protection of user data from unauthorized access and misuse.
- Data Encryption: Encrypted data storage and transfer practices safeguard sensitive information. This is vital for retaining user trust and complying with regulations.
- Access Controls: Limiting access to sensitive data is crucial. Only authorized personnel should have the capability to process or review tagged data, thus minimizing risks related to data exposure.
- User Rights: Automating tagging processes must align with user rights as stipulated by privacy regulations. This includes the right to access data, the right to rectification, and the right to be forgotten.