Analyzing BigQuery's Cost Structure for Effective Management
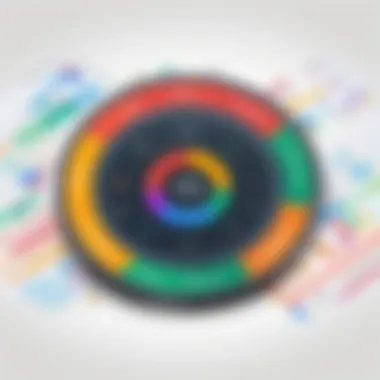
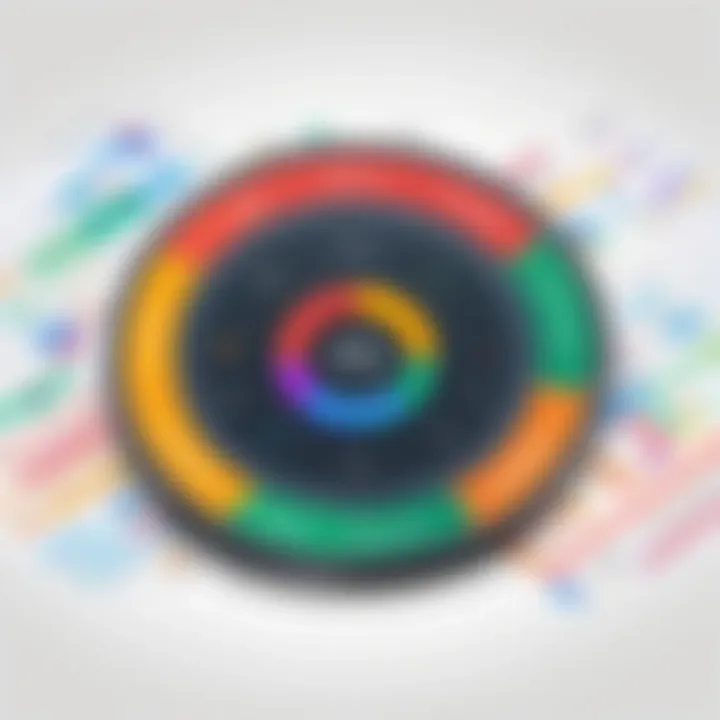
Intro
In a world inundated with data, the ability to store, manage, and analyze this information efficiently is a cornerstone of modern business strategies. Google BigQuery has emerged as a preeminent solution among enterprises seeking robust data warehousing capabilities. The strength of BigQuery, however, isn't solely in its feature set, but also in its multifaceted pricing model, which can at first glance appear intricate and daunting. Understanding how costs are structured is crucial for organizations hoping to harness this tool without breaking the bank.
This guide aims to deconstruct the various elements that contribute to the overall expenses incurred with BigQuery, laying out an at-a-glance perspective on what influences pricing. From storage fees to costs associated with running queries, we'll touch upon the aspects that matter to your budget and functionality. In doing so, tech-savvy individuals, business professionals, and IT experts will be empowered to make informed choices regarding their data management strategies, ultimately steering clear of unexpected costs.
An informed financial approach not only optimizes spending but can also enhance operational efficiency, transforming how organizations extract value from BigQuery's capabilities. Without further ado, let’s dive into the functionality of this data powerhouse.
Functionality
Overview of key features
BigQuery offers a host of features that cater to various data management needs, forging its reputation as a powerful player in the data warehousing arena. At its core, BigQuery is built for scalability and performance.
Major features include:
- Serverless architecture: This eliminates the need for managing infrastructure; users can run queries without worrying about the backend.
- Partitioned tables: This helps users organize their data into segments, which can enhance query performance and minimize costs.
- Machine learning capabilities: BigQuery’s integration with AI tools allows users to run predictive analytics within the platform, merging ELT capabilities with advanced analytics seamlessly.
- Geospatial analytics: It provides unique functions for analyzing geographic data, widening the applicability of analytics.
Beyond its features, the key question is how well BigQuery meets user needs. Many find the adaptability of the platform to be a standout characteristic.
How well the software meets user needs
BigQuery's design emphasizes flexibility, allowing it to cater to diverse project requirements without compromising ease of use. Its intuitive interface enables users, regardless of technical expertise, to execute complex queries and visualize data trends quickly. Moreover, the platform's asynchronous querying functionality gives users the freedom to handle more extensive datasets without being bogged down by latency issues.
Organizations that leverage BigQuery often report enhanced operational workflows. Users collaborating across teams can share data insights easily, meaning decisions are made promptly and based on solid data analyses. Being cloud-native ensures updates and new features roll out seamlessly, so users can always tap into the limitless potential of Google BigQuery.
This underpins the necessity of fully grasping the cost structure associated with these features, which we will explore next.
Intro to BigQuery Costs
Understanding the costs associated with Google BigQuery is crucial for anyone looking to harness its data analytics capabilities effectively. As businesses find themselves flooded with data, leveraging tools like BigQuery becomes a game changer. However, while the tool provides powerful functionalities, it also comes with its own pricing intricacies. This section lays the groundwork for deeper insights into how costs are structured, helping users navigate their budgeting processes with ease.
Overview of BigQuery
BigQuery is a serverless data warehouse solution provided by Google Cloud, designed to analyze massive datasets in a matter of seconds. Its architecture means that users don’t have to worry about managing infrastructure, server maintenance, or tuning performance. Instead, they can focus on what matters most—extracting insights from their data. This makes it a popular choice among companies of various sizes, from small startups to large enterprises.
The pricing model for BigQuery is multifaced, encompassing not just storage costs but also fees for queries and data egress. Understanding each component can help organizations not only leverage its capabilities but also keep costs in check. This understanding is vital, especially in a landscape where data can quickly translate into significant expenses if not managed properly.
Importance of Cost Management
Managing costs effectively in BigQuery is not merely a recommendation, it’s a necessity. When utilizing such a comprehensive tool, organizations can sometimes overestimate their budgetary requirements, leading to potential financial strain.
"Failing to plan is planning to fail."
The tools and resources available within BigQuery can become costly if left unchecked. By closely monitoring usage and understanding how different elements contribute to the overall cost, users can make informed choices that help mitigate unexpected expenses.
Key reasons why cost management is essential include:
- Optimizing Budget: Knowing the ins and outs of costs helps in optimizing the budget allocation. Doing so ensures that resources are used wisely.
- Forecasting Future Expenses: A true understanding of cost structure facilitates forecasting future expenses more accurately. This is particularly beneficial for long-term planning.
- Resource Management: Companies can manage consumption habits by tracking their costs closely, leading to smarter decisions regarding data storage and usage.
- Performance vs. Cost: Organizations may need to balance between the speed of queries and the costs associated with them, ensuring that they achieve efficiency without breaking the bank.
Together, these elements underscore the importance of a solid grasp of the BigQuery cost structure. By navigating this landscape with caution, organizations can position themselves to not only maximize the performance of their data analytics but also maintain fiscal responsibility.
Pricing Components
Understanding the pricing components of BigQuery is crucial for any organization aiming to harness data effectively. These components directly influence the overall expenditure, making it essential to grasp the intricacies involved. With various elements in play—such as storage, queries, and additional services—being aware of how each affects costs empowers users to craft a more budget-friendly data strategy. In essence, managing these pricing factors wisely can not only assist in cost allocation but also help in optimizing resource utilization.
Storage Costs
Storage costs form a significant part of BigQuery's pricing structure. In BigQuery, data storage isn't a flat fee; it fluctuates based on the volume of data stored and the type of storage used. Users have the option between active and long-term storage.
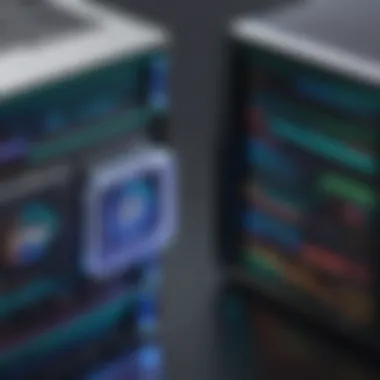
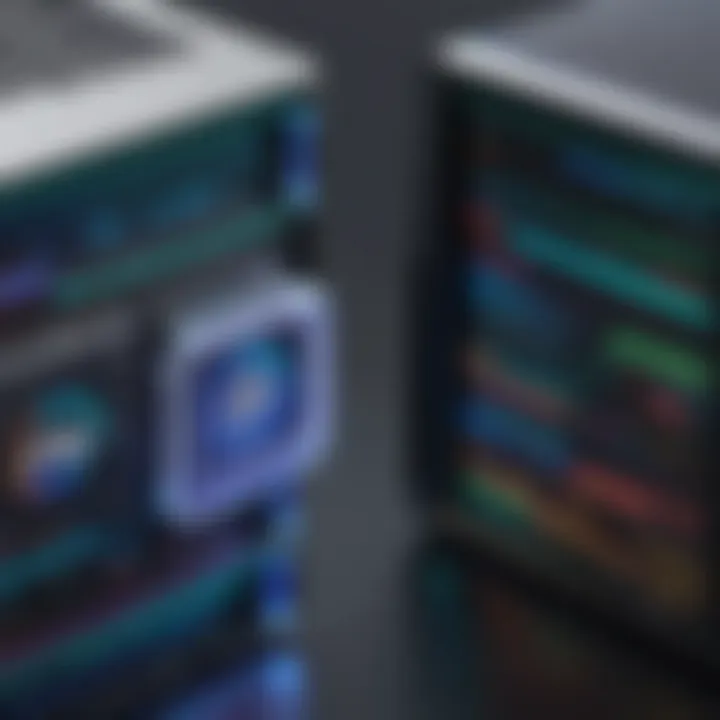
- Active storage is for data that is frequently accessed and updated. This is charged monthly based on the amount of data stored, so keeping a keen eye on unnecessary data can save bucks.
- Long-term storage applies to data that hasn't been modified for over 90 consecutive days, automatically reducing the storage cost for the aging data. This is a great incentive to retain historical data without breaking the bank.
Monitoring and managing storage usage can alleviate unexpected spikes in expenses. Setting retention policies ensures that outdated or unneeded data doesn't linger, saving both cash and keeping the data pool organized.
Query Costs
Query costs are another aspect of BigQuery pricing that cannot be overlooked. These charges are primarily based on the amount of data processed per query. Here’s where sophistication comes into play. For instance, simple queries may only touch a few kilobytes, while complex analytics might comb through terabytes of data.
To manage these costs effectively, a few strategies can be adopted:
- Partitioning and Clustering: Dividing datasets into more manageable chunks can make queries faster and diminish the data scanned.
- **Using Preview Features: Before executing an expensive query, utilize the preview feature to assess how much data will be processed.
Understanding the structures and behaviors of data can lead to better query design, which is not only more economical but also faster.
Streaming Insert Costs
Interest in real-time data has surged, and BigQuery accommodates this demand with streaming inserts. However, while the ability to analyze data as it arrives is appealing, it comes with costs tied directly to the volume of data streamed. Each gigabyte ingested incurs a fee, differing from batch processing, which charges based on data stored post-ingestion.
Organizations looking to utilize streaming should weigh the necessity against expenses. A balance of both streaming and batch processing might be required, ensuring that real-time insights do not lead to an astronomical bill in the end.
Data Egress Fees
Data egress fees are often a sticky subject among users, especially those who are new to BigQuery. Whenever data is pulled out of BigQuery into other Google services or external platforms, fees apply.
- Inter-region Transfers: In such transfers, costs can quickly accumulate, especially if data flows frequently between different regions.
- External Queries: If queries are run against external sources stored in services like Google Cloud Storage, understanding how egress can apply will help in anticipating future costs.
It’s advisable to map out data workflows and identify where data will be egressed to mitigate unnecessary costs.
Additional Service Costs
The landscape of BigQuery pricing encompasses various additional services as well. This might include features like machine learning capabilities through BigQuery ML, or data visualization tools integrated with BigQuery. Each of these services has its own pricing model that can affect the overall expenditure.
Some additional factors include:
- User Authentication: Integrations with identity management solutions may incur costs based on user counts.
- Data Share: Sharing datasets can give rise to network charges or additional fees depending on settings.
Understanding these supplementary service costs ensures holistic budget management and prevents shock expenses down the line.
In summary, awareness of these pricing components—storage, query, streaming inserts, egress fees, and additional service costs—not only paves the way for more informed decisions but also supports organizations in effectively budgeting their BigQuery spend.
Cost Calculation Examples
Understanding the cost calculation examples for Google BigQuery is essential for users looking to manage their data expenses efficiently. By breaking down potential use cases into concrete scenarios, businesses can align their data strategies with their budget. Cost calculation highlights how specific elements, such as the volume of data handled, the complexity of queries run, or the frequency of data insertions, directly impact the overall expenditure. Furthermore, grasping these calculations allows organizations to make informed decisions on whether to scale their operations or optimize their current processes. This insight is crucial, especially when managing data growth over time, as it influences both short-term spending and long-term strategy.
Use Case Scenarios
To appreciate the cost structure of BigQuery, consider various use case scenarios. For example, a retail company utilizing BigQuery to analyze sales trends may load daily transaction data into the system. When estimating costs, factors such as the total amount of data stored, frequency of queries, and the complexity of SQL commands must be taken into account. If this company runs a simple query to sum up daily sales, the costs will reflect the minimal compute resources consumed. On the other hand, a more complex query involving multiple joins and aggregations could push costs higher.
Understanding these scenarios aids businesses in projecting expenses based on realistic operational behaviors. Different sectors, be it retail, finance, or marketing, may have unique data challenges, and tailoring cost estimation models to those specific needs can yield more accurate forecasts.
Estimating Costs for Small Businesses
For small businesses, diving into BigQuery's cost structure could seem daunting at first. However, with thoughtful planning, estimating costs becomes more manageable. Generally, small firms do not handle colossal datasets initially, which can imply lower storage fees. For instance, if a small e-commerce site uses 1 TB of storage but runs only a handful of queries a week, the cost computation becomes straightforward:
- Storage cost: Assume $0.020 per GB, leading to approximately $20 per month for storage.
- Query cost: If each query processes 1 TB and the monthly usage is 5 TB in total, at $5 per TB, querying could tally up to $25.
In total, small businesses could expect a monthly bill around $45, a compromise that remains far less daunting than anticipated.
Estimating Costs for Enterprises
On the flip side, large enterprises grappling with vast datasets face a different challenge. Cost estimation in such environments often requires a nuanced understanding of inside metrics. Consider a multinational company collecting data from various branches worldwide. The sheer volume, combined with ongoing analytical processes like real-time reporting, necessitates careful calculation. Here, enterprises need to assess:
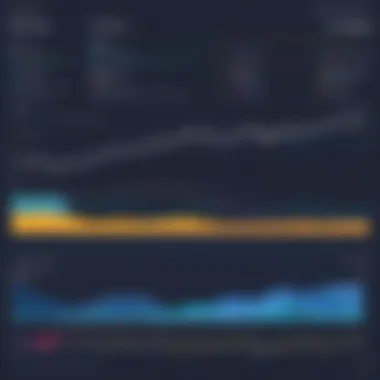
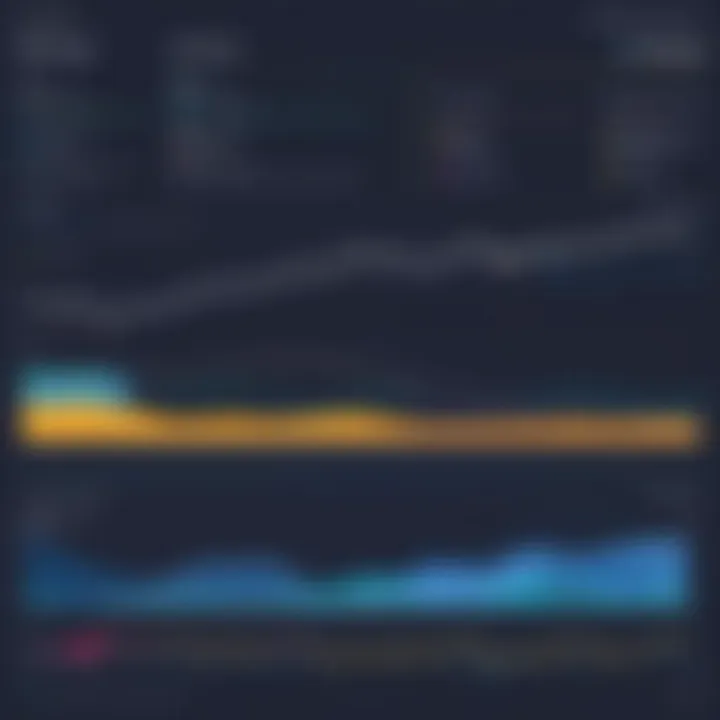
- Volume of Data: Total storage needed across various departments.
- Query Complexity: More intricate queries will incur higher costs.
- Regulatory Compliance: Ensuring data regulations can also add to costs if extra storage or management systems are required.
- Service Level Agreements: Firms may opt for reserved capacity to control costs further, which can save money but requires upfront commitment.
A multifaceted approach to estimating costs in enterprises proves vital. Meetings among IT, finance, and operational teams often yield more reliable data insights and cost predictions. Investing time upfront in understanding BigQuery’s cost structure pays off, preventing budget surprises down the line.
In summary, whether a small business or a large corporation, developing an accurate understanding of BigQuery's cost calculations fosters better budget management and informed decision-making regarding data strategy.
Cost Optimization Strategies
In the realm of BigQuery, understanding costs is an ongoing challenge and opportunity for enhancement. Cost Optimization Strategies serve as crucial methodologies for organizations to minimize unnecessary expenditures while maximizing the utility of their data operations. Given the increasingly competitive landscape, even the smallest adjustments to cost structures can lead to significant savings. Let's explore specific strategies that can be employed.
Data Lifecycle Management
Managing data effectively throughout its lifecycle is a vital piece of the cost optimization puzzle. Data lifecycle management (DLM) implies understanding the journey of data from creation to obsolescence. Organizations need to assess the necessity of retaining data. The longer data stays in the system, the higher the costs incurred for storage.
In this strategy, implementing tiered storage can be incredibly beneficial. Using less expensive storage options for infrequently accessed data can dramatically cut costs. For instance, consider moving archived data that is rarely queried into a cheaper storage class. Some key points include:
- Identify usage patterns: Regularly monitor which datasets are frequently queried. This analysis helps in deciding what data can be moved or archived.
- Automated policies: Set up automated rules that transition data based on its age or access frequency.
- Cost analysis: A detailed study of storage costs versus access frequency can prevent unnecessary expenses.
Query Optimization Techniques
Query optimization is another facet where precision can lead to lowered costs. Inefficient queries not only waste time but also rack up usage fees in BigQuery. Well-constructed queries can drastically reduce the amount of data processed, which translates to savings. Here are some strategies:
- Select only necessary columns: Instead of using a wildcard like , specify the columns you need. This minimizes the volume of data processed.
- Use partitioned tables: Break your data into logical segments based on time or other criteria to speed up queries and reduce scanning costs.
- Leverage clustering: Similarly, clustering can allow BigQuery to narrow down data more efficiently, thus reducing the amount of data processed during a query.
Additionally, monitoring query performance and employing techniques like caching can drastically improve efficiency. The less data you query, the less it costs. A common mistake is overlooking optimization; consistently reviewing query performance is a must.
Leveraging Reservations
Google BigQuery offers a reservation system that enables organizations to purchase slots for dedicated query processing. This effectively pre-pays for compute time, which can lead to decreased costs if utilized properly. Leveraging reservations can free you from the whims of on-demand pricing and grant access to a predictable cost structure.
- Assess your usage patterns: If your organization frequently queries large datasets or runs periodic batch jobs, using reserved slots may lead to substantial savings.
- Flexible commitments: BigQuery allows for flexibility in commitment periods, ranging from short-term to long-term. Assess your project's timeline to choose the most economical option.
- Slot pool management: Take advantage of the shared slot pools for smaller teams or projects. This can maximize efficiency without overcommitting financial resources.
"By employing these cost optimization strategies, organizations can take charge of their BigQuery expenses while enhancing their data management capabilities."
Incorporating these advanced strategies into your BigQuery utilization can not only boost efficiency but can also keep costs manageable. Keeping a keen eye on how data is handled and executing precise queries will lead to more cost-effective data operations.
Comparative Analysis with Other Solutions
In today's rapidly evolving data landscape, organizations are faced with a myriad of options for data warehousing solutions. This comparative analysis section serves as a compass for businesses looking to navigate the intricate waters of data management costs. By contrasting BigQuery with its prominent competitors, organizations can gain insights into which solution presents the best value for their specific needs.
Understanding the cost structure of various data management tools like BigQuery, Redshift, and Snowflake is crucial for smart budgeting. A thorough analysis assists professionals in identifying potential savings and helps guide their decisions on infrastructure investments. Essentially, this section not only explains cost metrics but also provides contextual insights that empower business leaders to choose wisely within their ecosystem.
Cost Comparison: BigQuery vs. Redshift
When considering BigQuery against Amazon Redshift, organizations ought to focus on several pivotal pricing factors.
- Storage Fees: BigQuery employs a pay-per-query model, meaning its storage costs can accumulate based on how data is queried and accessed. Conversely, Redshift utilizes a more traditional approach with fixed costs tied to provisioned resources. Companies must evaluate their data consumption patterns to determine which model makes financial sense.
- Scaling Flexibility: Consider Elasticity. BigQuery auto-scales based on user demand, allowing for it to handle varying workloads without reservation. Redshift, on the other hand, may require upfront capacity planning and additional costs if resource needs evolve unexpectedly.
- Query Performance Costs: BigQuery charges per query processed, which could entice companies with sporadic usage. Redshift's costs hinge on the sustained load, which could become financially burdensome for businesses with fluctuating workloads. This factor opens the door for companies to maintain tighter budgets during periods of reduced usage.
"Choosing a data warehousing solution goes beyond initial costs; it requires a discerning eye on total cost of ownership."
Cost Comparison: BigQuery vs. Snowflake
The financial dynamics between BigQuery and Snowflake reveal nuanced distinctions that can shape organizational strategies.
- Pricing Models: BigQuery excels in its serverless approach, ensuring that you only pay when you process queries. Snowflake operates under a separate storage and compute pricing model, allowing organizations to scale each independently. This often leads to significant cost efficiency when managed strategically.
- Data Storage and Retrieval Costs: Snowflake offers tiered storage options which can adapt based on retrieval needs, with a focus on minimizing costs for rarely accessed data. While BigQuery allows for controlled costs tied directly to usage, businesses must watch out as frequent queries can quickly inflate expenses if not optimized.
- User Experience and Administrative Expenses: BigQuery's straightforward infrastructure could imply lower administrative overhead, while Snowflake’s sophisticated features might require a bit more oversight to optimize spend effectively.
For IT professionals and business leaders, these comparative assessments illuminate the financial landscape of data warehousing solutions. It’s essential to weigh out these factors carefully, taking into account not only current needs but also future growth and change. By identifying the intricacies within pricing models, businesses stand to bolster their data strategies without compromising their budgets.
Real-world Success Stories
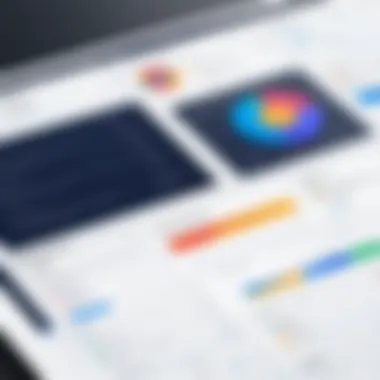
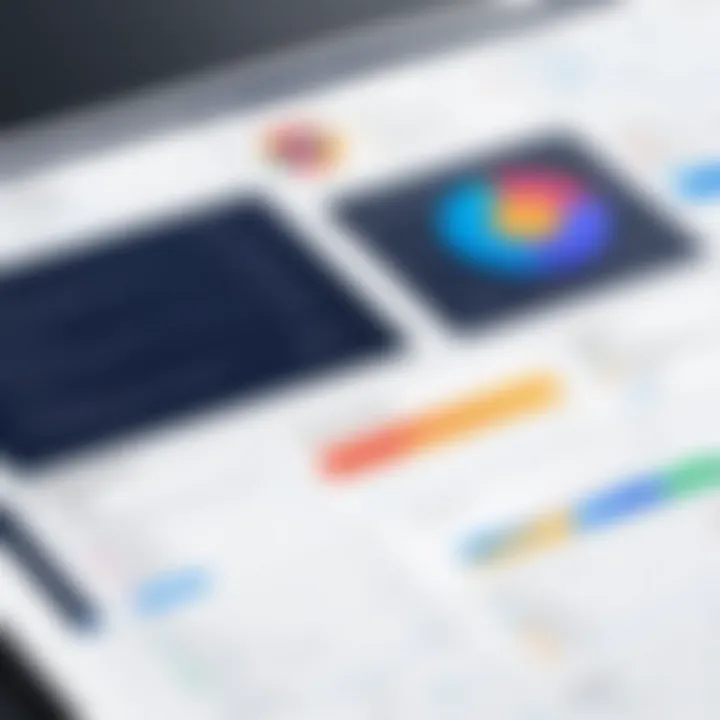
Understanding actual implementations and the tangible benefits that organizations have gained from using BigQuery can provide crucial insights for prospective users. Real-world success stories serve as powerful reminders that BigQuery's cost structure not only aids in financial planning but also enhances operational efficiencies. As businesses adapt to ever-evolving data demands, these case studies highlight how savvy investment decisions in data storage and analysis can lead to significant financial and strategic advantages.
Case Study: Small Business Efficiency
Imagine a small accounting firm that traditionally processed data through standard databases. As the business grew, so did its client base and, consequently, the amount of data. The firm found themselves in a bind: slow query times and escalating costs associated with their on-premises solutions could strain both budgets and timelines.
Upon adopting BigQuery, the firm experienced a rapid transformation. They discovered several key efficiencies:
- Cost-Effective Pricing: Initially skeptical, the firm found that on-demand pricing offered by BigQuery allowed them to pay only for the queries they actually ran. This was a game-changer, eliminating the need for hefty upfront investments in hardware that would have been underused during quieter periods.
- Faster Insights: Query times dropped dramatically, allowing the accountants to retrieve critical reports in mere seconds rather than hours. This reliability in timing created a competitive edge as they could provide timely recommendations to clients.
- Scalable Solutions: As the firm grew, its data needs evolved. Thanks to BigQuery’s scalability, the firm could seamlessly scale its storage capacity without the usual concerns of managing physical servers or incurring downtime.
In this instance, leveraging BigQuery not only resulted in significant cost savings but also streamlined operations, aligning perfectly with the small business's goal of enhancing service delivery while keeping overhead low.
Case Study: Large Corporation Governance
Now consider a large corporation that operates in the healthcare sector with a vast amount of sensitive data. This corporation was juggling compliance with regulatory standards, managing patient data while simultaneously needing to analyze this data for insights that could lead to improved patient outcomes. The complexity of their data infrastructure posed a major hurdle in both governance and operational efficiency.
Upon transitioning to BigQuery, they encountered multiple benefits that transformed how they approached data management:
- Integrated Compliance: The company was able to efficiently handle sensitive data with enforced security protocols readily available in BigQuery. This compliance with standards like HIPAA not only saved potential fines but also improved trust with stakeholders.
- Real-time Analytics: With access to real-time data analytics, the corporation could respond promptly to emerging trends and issues within patient care, thus enhancing decision-making processes. This was crucial, especially in moments demanding rapid action, like during healthcare crises.
- Data Collaboration: Centralizing their datasets in BigQuery allowed cross-departmental teams to collaborate closely. Thanks to BigQuery’s simplicity and user-friendly nature, even non-technical staff could generate queries and derive insights without overwhelming IT teams.
By adopting BigQuery, this large organization not only streamlined its governance processes but also advanced its mission of providing quality healthcare in a responsible and efficient manner. The transition turned into a clear demonstration of how appropriate data management investments can yield fruitful returns.
"Transforming data into insights can create a ripple effect that enhances the entire organization’s efficiency and compliance."
These real-world success stories vividly illustrate that understanding BigQuery's cost structure can lead to strategic plans that align business objectives with efficient data management. With a thoughtful approach to cost management, users can unlock the true potential of their data-driven initiatives.
Frequently Asked Questions
Knowing the common inquiries can guide decision-making processes for businesses and tech-savvy individuals aiming to optimize spending.
Common misconceptions about BigQuery costs
One prevalent misconception is the belief that using BigQuery is prohibitively expensive for all use cases. While there may be scenarios where costs can escalate, understanding the pricing model shows it can be quite flexible. For instance, some might think they always pay exorbitantly when running queries. However, BigQuery’s pricing varies based on factors like data volume and query complexity. In some cases, users can achieve significant savings by optimizing their queries effectively.
Another misconception revolves around storage costs. Many assume that all storage in BigQuery incurs the same fee, but that isn’t the case. There are different tiers of storage, such as active storage and long-term storage, which vary in cost structure. This differentiation means users can leverage strategies to minimize expenses further by managing data efficiently.
Additionally, some users worry about hidden costs associated with running queries or exporting data. The reality is that BigQuery provides clear documentation and tools that allow users to calculate expected costs before execution, leading to better financial planning.
How to predict future costs?
To predict future costs in BigQuery effectively, organizations should consider their unique usage patterns. A thorough analysis of previous months’ queries and storage usage can provide valuable data. Tracking metrics such as the amount of data processed per query and the types of queries commonly run gives insights into potential future expenses.
Utilizing Google Cloud’s cost management tools can further aid in forecasting. Users can set budgets and alerts that notify them when they approach specified spending limits, which is an excellent way to avoid budget overruns.
Additionally, adopting a careful approach to data management is essential. By reviewing query histories and identifying opportunities for optimization, future costs can be reduced significantly.
In essence, thorough planning and continual monitoring of usage patterns are essential for predicting and managing future costs. Organizations should not treat this as a one-time job. Regular reevaluation is key to adapting to usage changes, helping to keep expenses in check.
End
Understanding the cost structure associated with Google BigQuery is paramount for organizations that seek to leverage this robust data warehousing solution. The topics discussed in this article offer a foundational understanding of how costs accumulate, which is crucial for sound financial planning.
When diving into the nitty-gritty of BigQuery’s pricing model, businesses can pinpoint areas where they can save money. For instance, being aware of how storage and query costs work can lead to more strategic data management decisions. This knowledge empowers users to identify potential pitfalls and capitalize on opportunities for cost optimization, thus ensuring that their dataset management aligns with their budget constraints.
The importance of this conclusion rests on several key points:
- Informed Decisions: Having a comprehensive understanding of cost structures helps organizations make educated choices about their usage of BigQuery.
- Efficiency Boosts: By knowing how to manage costs, companies can invest more resources in data analysis rather than in unexpected expenses.
- Predictability: Understanding future costs becomes attainable when organizations can anticipate their data needs and associated expenses, making it easier to allocate budgets.
- Strategic Planning: Equipped with knowledge about cost components, businesses can plan for scaling their operations without a steep learning curve regarding expenses.
Ultimately, effective cost management in BigQuery leads to fiscal responsibility while maximizing the potential of data-driven decision-making. The journey through the various components of costs, optimization strategies, and real-world applications not only enriches the narrative of understanding BigQuery's pricing but also fortifies a company’s strategic advantage in their operational expenditure.
Key Takeaways on Cost Management
- Analyzing Costs Regularly: Always audit usage against expected costs. Regularly review which services you utilize and ensure they align with your budget goals.
- Optimize Queries: Efficiently structure your SQL queries to minimize unnecessary scans and usage. Consider using partitioning and clustering for better performance and reduced costs.
- Monitoring Data Egress: Being cautious about data transfer costs can save funds that would otherwise be unnecessarily expended on data egress fees.
- Leverage Reservations: If your usage pattern is consistent, consider using flat-rate pricing through on-demand reservation to reduce query costs significantly.
"Knowing is half the battle." This adage rings particularly true in the realm of BigQuery cost management. The more you know about how costs accrue and how to manage them, the better equipped you are to strategize.
By keeping these points in mind, organizations can navigate the financial ramifications of BigQuery with a clear head and a robust strategy.