Comprehensive Review of Statistical Forecasting Techniques
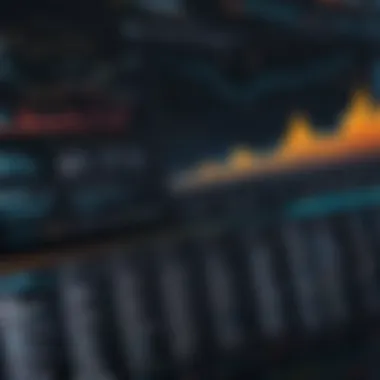
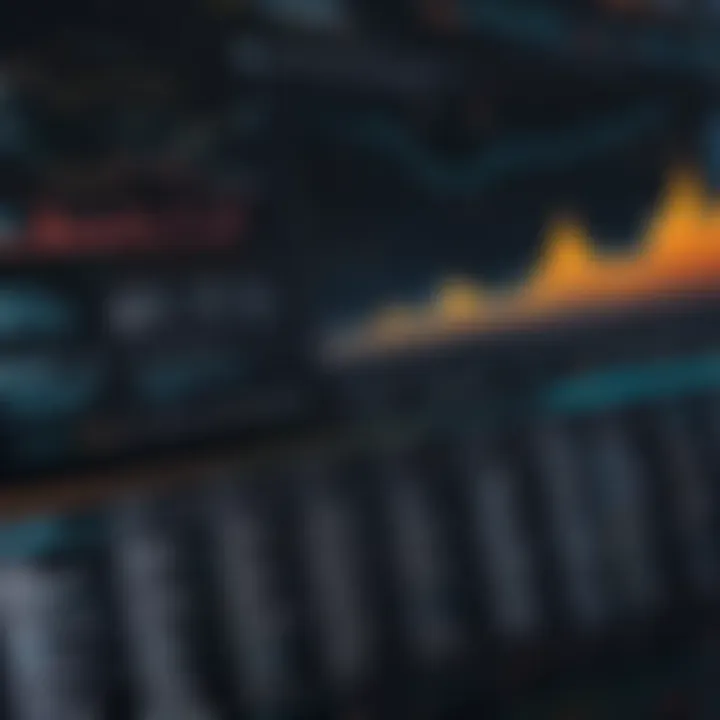
Intro
Statistical forecasting plays a vital role in decision-making across various industries. At its core, it serves as a reliable guide for organizations seeking clarity in uncertain environments. As rapidly changing markets throw up challenges, businesses are increasingly leaning on statistical methods to construct a roadmap for the future. The core of statistical forecasting lies in its ability to transform raw data into predictive insights, steering organizations toward informed strategies.
What makes statistical forecasting even more significant today is the sheer volume of data businesses collect. From sales figures to consumer behavior patterns, the data landscape is vast and complex. However, without effective analysis and interpretation, this valuable information can become a burden rather than an asset. Herein lies the value of employing statistical forecasting methods: they offer structured approaches for analyzing trends, detecting patterns, and creating projections that can shape decision-making processes.
In this article, we delve deeper into the realm of statistical forecasting. We will dissect various methodologies, provide a glimpse into theoretical concepts, and showcase real-world applications. Additionally, the article will touch on the implications of accurate forecasting in sectors like finance, retail, and even healthcare. As we navigate through case studies and advancements, readers will gain a comprehensive understanding of how statistical forecasting can be harnessed effectively in diverse scenarios.
Preamble to Statistical Forecasting
Statistical forecasting serves as a cornerstone of decision-making in a multitude of sectors. It involves the systematic use of data to predict future events or trends, allowing organizations to allocate resources wisely and strategize accordingly. Without the benefit of reliable forecasting, businesses risk venturing into uncertain territories, where they might end up missing opportunities or encountering unexpected pitfalls. As we dive into this subject, we'll explore essential elements, the spectrum of benefits, and the subtle yet crucial considerations associated with it.
Definition and Importance
At its core, statistical forecasting is the practice of making informed predictions based on historical data and statistical methods. It encompasses various techniques that range from simplistic calculations to complex models that integrate multiple variables. The importance of statistical forecasting cannot be overstated. For instance, in the retail sector, accurate predictions regarding customer demand can mean the difference between surplus stock gathering dust and meeting customer needs promptly. In the realm of finance, it can guide investment strategies to maximize returns while minimizing risks. By understanding and leveraging statistical forecasting, organizations can make evidence-based choices, ensuring a competitive edge in todayās fast-paced environment.
Historical Context
The roots of statistical forecasting trace back several centuries, intertwining with the evolution of mathematics and statistics. Initially, societies relied on observational techniques and rudimentary calculations to predict agricultural yields or seasonal changes. Over time, with the advent of statistics as a formal discipline in the 18th century, methods became more sophisticated. Early 20th-century innovations, such as the development of econometrics, paved the way for advanced forecasting models used today. These advancements underscore how statistical forecasting has transformed from a rudimentary practice into a complex field that drives critical business and economic decisions. As we delve deeper, we'll examine the pivotal moments that have shaped its development and how past practices inform contemporary approaches.
Foundations of Statistical Forecasting
Understanding the foundations of statistical forecasting is like laying the groundwork for a sturdy structure. Without a solid base, the subsequent processes lack stability and reliability. These foundations make it possible to accurately predict future outcomes, which is fundamental in many fields like finance, marketing, and operations. Not only do these principles guide the forecasting efforts, but they also help in tailoring methods to suit specific needs and contexts.
Key Principles
At the core of statistical forecasting lies a few key principles that guide analysts in their quest for accuracy and reliability. These principles include:
- Understanding Variability: Each dataset comes with its own quirks and variations. Recognizing this variability is crucial. It helps forecasters to differentiate between random noise and meaningful patterns.
- Past as Prologue: Statistical forecasting relies heavily on historical data. By analyzing previous trends, forecasters can derive insights that are predictive of future outcomes. This concept is summed up well in the adage, "history tends to repeat itself."
- Quantifying Uncertainty: Forecasting is not about ultimate truths; it's about probabilities. Models should account for uncertainty, presenting not just single point estimates but a spectrum of possible outcomes.
To put it simply, understanding these principles is essential for anyone involved in the field. Skills in statistical reasoning, data interpretation, and critical thinking are crucial to translate raw data into actionable insights.
Data Requirements
The effectiveness of statistical forecasting is systemically linked to the quality and type of data used. Like trying to bake a cake without eggs, making predictions without proper data is unlikely to yield great results. In statistical forecasting, there are specific data requirements that forecasters must consider:
- Volume and Variety: Forecasting needs a significant amount of data ranging from sales figures to economic indicators. The varied nature helps capture different facets of the issue being analyzed.
- Historical Data: Access to historical data is paramount. This data serves as the bedrock on which predictive models are constructed. Analysts often look for at least three years of data for robust prediction.
- Timeliness: The currency of the data plays a critical role, especially in rapidly changing industries. Real-time data may present advantages over outdated information, which might no longer be relevant.
- Data Cleansing: Before analysts can work their magic, data must be cleansed to remove inconsistencies. This includes correcting errors, filling gaps, and normalizing formats.
"In statistical forecasting, the quality of your input directly influences your output."
In sum, the foundations underpin the entire forecasting process. An understanding of these principles and a commitment to data integrity go a long way in yielding credible and actionable forecasts. Without these elements, forecasting can easily turn into a wild guess, leading organizations astray.
Types of Statistical Forecasting Methods
Understanding the types of statistical forecasting methods is crucial for anyone involved in the world of prediction and data analysis. These methods can significantly impact decision-making processes across various fields from business to finance. By choosing the appropriate forecasting technique, organizations can glean insights that drive strategic actions and enhance operational efficiency. Here, we explore two main approaches: qualitative and quantitative methods, while also diving deeper into specific techniques such as time series analysis and causal models.
Qualitative vs. Quantitative Methods
When we talk about forecasting methods, it often boils down to two distinct categories: qualitative and quantitative. Both serve unique purposes in statistical forecasting, and understanding these differences can make a world of difference in application.
Qualitative Methods rely heavily on subjective judgment or intuition rather than numerical data. They are useful when historical data is sparse or nonexistent. For a firm launching a new product, qualitative methods like expert opinions or focus groups can provide invaluable insights into consumer tendencies. However, their subjective nature can lead to inconsistencies, making it not always the best option for data-driven decision-making.
On the other hand, Quantitative Methods utilize numerical data to guide predictions. They are often viewed as more reliable since they are based on statistical calculations. Forecasting through historical sales data, customer statistics, or economic indicators can offer a clearer picture of future trends. Nevertheless, these methods can sometimes overlook external factors, making it vital to choose wisely according to the context.
Time Series Analysis
Time Series Analysis is a quantitative method that focuses on analyzing data points collected or recorded at specific intervals over time. It unveils patterns and helps in predicting future values based on past observations, proving to be particularly advantageous for businesses monitoring their performance metrics.
Trend Analysis
Trend Analysis is a subset of time series analysis that aims to identify general directions in data trends over a long period. For instance, perhaps a retailer notices a steady increase in sales during the holiday seasons over the years. By employing trend analysis, they can project future sales, which helps in inventory planning and marketing strategies. The key characteristic of trend analysis is its focus on long-term movements, making it a popular choice for businesses wanting to understand emerging patterns. However, a downside is that it might overlook short-term volatility that could be critical.
Seasonal Decomposition
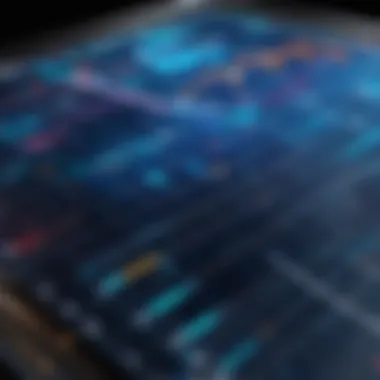
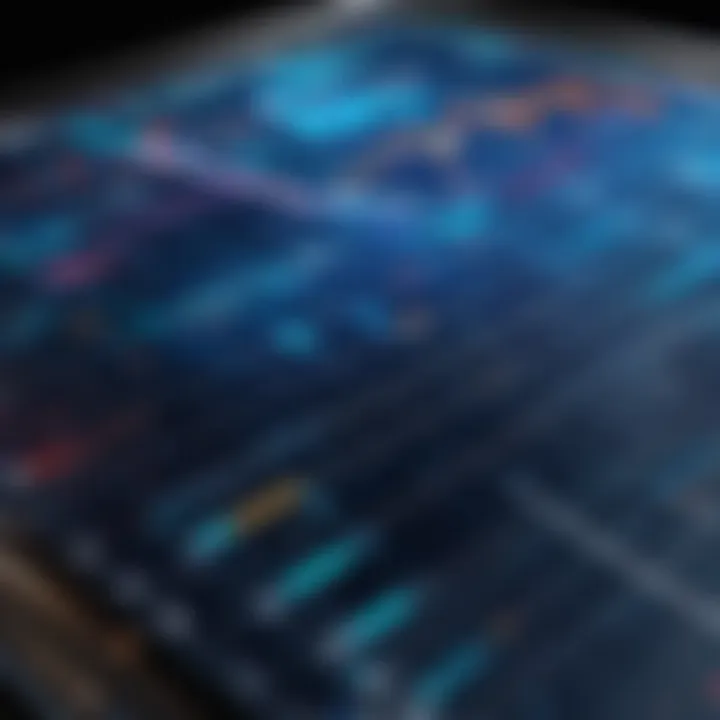
On the other hand, Seasonal Decomposition divides data into its underlying components: trends, seasonality, and residuals. For instance, a clothing company could utilize this method to identify how seasonal factors impact their sales at different times of the year. This insight allows them to adjust their production and marketing efforts accordingly. One key characteristic that makes seasonal decomposition beneficial is its ability to pinpoint regular fluctuations, which can help businesses allocate their resources effectively. However, a common disadvantage is its complexity and the potential misinterpretation of seasonal factors that can lead to wrong forecasting.
Causal Models
Causal Models delve into how variables are interconnected and how one influences another. This type of analysis helps in understanding the relationship between dependent and independent variables, offering a rich layer of predictive capability.
Regression Analysis
Regression Analysis is one of the most common causal models in forecasting. It quantifies the relationship between a dependent variable and one or more independent variables. For example, a marketing team might employ regression analysis to assess how advertising spend influences sales figures, providing them with quantitative targets for future campaigns. A strong point of regression analysis is its capacity to include multiple factors at once. However, it demands high-quality data, and the potential for overfittingāwhere the model describes random error rather than the relationship itselfācan pose a challenge.
Explanatory Variables
Explanatory Variables are key in establishing a cause-and-effect relationship in forecasting. They help to explain variations in the dependent variable, offering insights into the underlying factors that might affect behavior. For business professionals, leveraging explanatory variables can lead to a more comprehensive understanding of the marketplace dynamics. As a disadvantage, however, it can introduce complexity and requires careful consideration to avoid drawing incorrect conclusions from seemingly correlated data points.
In summary, understanding the various statistical forecasting methods enables professionals to effectively harness data insights. Whether itās through qualitative measures or more rigid quantitative analyses, employing the right method can significantly influence decision-making and strategic planning in diverse fields.
Tools for Statistical Forecasting
Understanding the various tools available for statistical forecasting is crucial for any professional looking to harness the power of data. These tools not only aid in the prediction of future trends but also enhance the decision-making process across diverse sectors. With the right tools at oneās disposal, organizations can analyze past data, identify patterns, and provide actionable insights that can steer strategic initiatives.
When considering tools for statistical forecasting, one must evaluate several factors:
- Ease of use: How intuitive is the interface?
- Flexibility: Can the software adapt to various forecasting methods?
- Integration capacity: Does it seamlessly integrate with existing systems?
Utilizing these tools can significantly increase the reliability of forecasts, ultimately impacting the bottom line positively.
Software Solutions
Comparison of Forecasting Software
The comparison of forecasting software is an essential component in understanding which solution aligns best with specific organizational needs. Not all software packages are created equal, and the differences can be substantial depending on their functionalities. Key characteristics of various software might include pricing structures, data input requirements, and analytical capabilities.
One of the most beneficial aspects of this comparison is its ability to highlight how certain software options are particularly suited for specific industries or use cases. For instance, some tools cater primarily to retail forecasting by incorporating consumer behavior patterns. From statistical packages like R and Python libraries to specialized software like Forecast Pro, each has its unique feature set designed to tackle distinct forecasting challenges.
However, a noteworthy downside is that the niche features required may come at additional costs or may involve a steep learning curve for new users. It's important to weigh these factors according to your teamās needs and expertise.
User Reviews and Insights
In the realm of statistical forecasting, user reviews and insights play a pivotal role in shaping perspectives about various software solutions. Users often provide firsthand accounts that can illuminate the strengths and weaknesses of a tool. This feedback is invaluable for organizations seeking to make informed decisions about software purchases.
The key characteristic of these reviews is their practical relevance; they often highlight real-world applications and the outcomes involved. By assessing user experiences, potential buyers can gain insights into how these tools operate, revealing common pitfalls or exceptional benefits.
A unique feature of user reviews is their ability to direct attention to issues that may not be covered in marketing materials, such as customer service responsiveness or software scalability. These insights can significantly affect the selection process, as a highly-rated product with excellent support can lead to smoother implementation and higher satisfaction.
Integration with Data Analytics
The relevance of integration with data analytics cannot be overstated. The ability for forecasting tools to integrate smoothly with existing data analytics platforms is fundamental for maximizing the efficacy of forecasting efforts. When tools can communicate with analytics systems, organizations can enhance the granularity of their data analysis.
Here are several considerations regarding integration:
- Data Sources: Can the tool accommodate various data formats?
- Collaboration: How well does it facilitate teamwork across departments?
- Real-Time Data: Does it allow for live data feeds that keep forecasts updated?
Integrating forecasting software with data analytics fosters a holistic view of data, allowing teams to refine their forecasting processes continually. Such synergy leads to improved accuracy and focuses on real-time adaptations to changing market conditions.
Overall, the right tools for statistical forecasting can significantly enhance decision-making capabilities across various business functions. Choosing the appropriate software and ensuring seamless integration with data analytics requires careful consideration and thorough evaluation.
Applications of Statistical Forecasting
Statistical forecasting plays an essential role in interpreting data and informing critical decisions across a variety of sectors. The accuracy of predictions can significantly influence operational efficiency, resource allocation, and strategic planning. Concepts like regression models and causal analysis offer businesses a blueprint to navigate markets and consumer behaviors. This section delves into three major domains where statistical forecasting excels: business and economic forecasting, supply chain management, and financial analysis.
Business and Economic Forecasting
In the realm of business, forecasting isn't just a crystal ball; itās the bedrock of informed decision-making. Companies use statistical forecasting to predict market trends, customer demand, or even the economic climate. By analyzing historical data and identifying patterns, organizations can anticipate changes in consumer preferences and adjust their strategies accordingly.
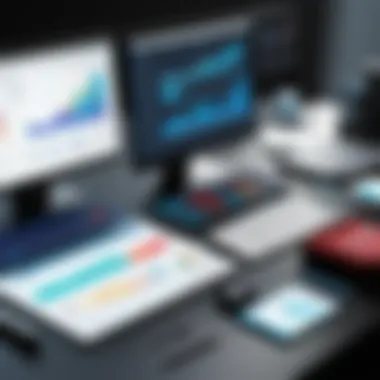
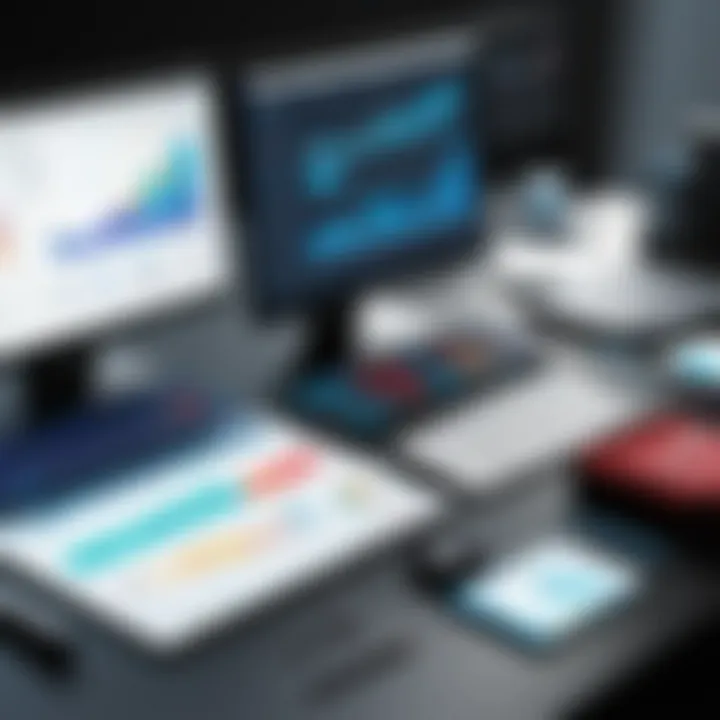
- Consumer Behavior Prediction: For instance, retail giants utilize statistical models to determine purchasing trends, allowing them to manage inventory and customize marketing strategies effectively.
- Economic Indicators: On a broader scale, economic forecasting assesses indicators like GDP growth, unemployment rates, and inflation. Understanding these variables provides businesses with a factual basis for entering new markets or developing products.
Incorporating statistical forecasting into business practices encourages proactive rather than reactive approaches, ultimately driving profitability.
Supply Chain Management
For supply chain professionals, managing logistics efficiently is paramount. Statistical forecasting allows organizations to maintain optimal stock levels and anticipate fluctuations in demand. By leveraging methodologies like time series analysis, businesses can forecast product demand with astonishing precision.
- Inventory Optimization: Accurate forecasts help prevent overstock and stockouts, which can hamper operations. If a company misjudges demand, either they waste resources or miss sales opportunities.
- Seasonal Trends: Industries often see seasonal demand spikes. A clothing retailer, for example, can predict sales increases around holidays by studying past performance statistics, ensuring theyāre stocked for busy periods without excess post-season inventory.
Thus, statistical forecasting is a critical tool for enhancing efficiency and reducing costs in supply chain management.
Financial Analysis
Lastly, financial analysts rely heavily on statistical forecasting to project revenues, expenses, and profitability. Financial forecasting uses a blend of qualitative and quantitative approaches, equipping analysts to make sound investment decisions.
- Risk Assessment: It helps in evaluating potential risks and returns of investment opportunities, allowing investors to pivot strategies in a fluctuating marketplace.
- Budget Planning: By employing regression analysis on historical financial data, companies can predict future performance, enabling them to set realistic targets and budgets.
In this way, statistical forecasting empowers financial analysts to navigate uncertainties, optimize portfolios, and enhance financial stability.
"Accurate forecasting is not just an analytical tool; it's a strategic imperative in the fast-paced business environment."
Ultimately, by embracing statistical forecasting in these applications, organizations not only enhance their decision-making processes but also strengthen their competitive edge.
Challenges in Statistical Forecasting
Statistical forecasting is a powerful tool in any decision-makerās arsenal. However, it is no bed of roses. A myriad of challenges lurks in the shadows, and to harness the true potential of statistical forecasting, one must be fully aware of these stumbling blocks. More than mere speed bumps, these challenges can derail even the most sophisticated predictive models, leading to suboptimal decisions. As we dig into the nitty-gritty of statistical forecasting, understanding these challenges is not just beneficial; it's essential.
Data Quality Issues
Data quality issues stand as formidable challenges in statistical forecasting. Poor-quality data can arise from a multitude of sources. Whether due to human error in data entry or inherent flaws in data collection methods, the consequences can be catastrophic. When the foundation of a forecast is shaky, no amount of complex modeling can salvage it.
To illustrate, consider a retail company that plans to forecast sales for its new line of products. If the historical sales data used for the forecast contains numerous inaccuraciesālike incorrectly inputted figures or missing entriesāthe forecasts will reflect these discrepancies, ultimately leading to stock shortages or overstock situations.
In addition to human error, external pressures like changing market dynamics can severely impact the quality of data. When companies fail to adjust their models to account for these shifts, their forecasts might become irrelevant. Regular audits of data sources, validation processes, and continual updates are vital in maintaining data integrity, ensuring that the foundation for forecasting remains robust.
Model Selection and Overfitting
Another hurdle that often trips up forecasters is model selection and overfitting. The world of statistical methods is vast, brimming with different models each tailored for specific scenarios. The challenge lies in choosing the right model that aligns with both the nature of the data and the forecasting goals.
Overfitting occurs when a model is excessively complex, capturing noise rather than the underlying trends. This inclination to fit too closely to historical data can make future predictions unreliable. For instance, a machine learning model that accounts for every slight variation in historical data might impress at predictive accuracy on training sets, but once deployed on new data, it may falter.
To combat this challenge, forecasters often turn to cross-validation and other techniques that assess a modelās performance using unseen data, thereby ensuring that it holds up under real-world conditions. Striking a balance between capturing significant trends without falling into the overfitting trap is critical yet often challenging.
External Factors and Uncertainty
The landscape of statistical forecasting is often clouded by external factors and uncertainty. Market dynamics, socio-economic factors, and unforeseen events like natural disasters or pandemics can throw a wrench into even the most well-planned forecasts. For example, the COVID-19 pandemic drastically altered consumer spending patterns, proving that external shocks can outpace traditional forecasting methods, rendering them nearly obsolete in the face of rapid change.
Moreover, the uncertainty surrounding these external factors makes it all the more challenging to devise reliable forecasts. When predicting outcomes, forecasters must account for not just historical data but also potential future disruptions. This often involves scenario planning and sensitivity analysis, allowing for a wider range of outcomes and enhancing preparedness.
In summary, addressing these challenges is not merely an academic exercise; it is crucial for any organization relying on statistical forecasting. A nuanced understanding of data quality, model selection, and the impacts of external uncertainty not only enhances the accuracy of forecasts but also fortifies the decision-making processes that hinge on these predictions.
"To ignore the challenges in statistical forecasting is to flirt with uncertainty; to embrace them is to wield precision."
By acknowledging and actively working through these hurdles, businesses can transform statistical forecasting from a daunting task into a strategic advantage.
Recent Trends in Statistical Forecasting
The field of statistical forecasting is undergoing a significant transformation, driven by technological advancements and the increasing volume of data generated in numerous sectors. Keeping an eye on recent trends is crucial for professionals aiming to leverage forecasting methods effectively. This section delves into two prominent trends: the integration of machine learning and the rise of big data analytics. Both trends offer new, innovative pathways for enhancing forecasting accuracy and efficiency, making them essential topics for any tech-savvy professional or business decision-maker.
Machine Learning Integration
Machine learning has become a game changer for statistical forecasting. Unlike traditional methods that often rely on predefined models, machine learning algorithms can learn patterns from vast datasets and improve predictions over time. This adaptive nature is particularly valuable in environments where data is continually evolving.
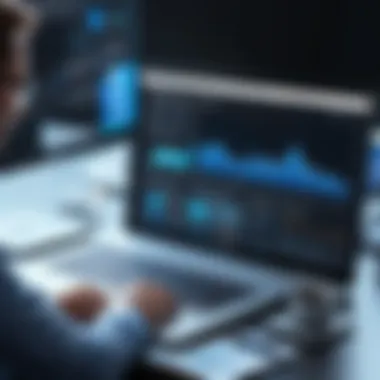
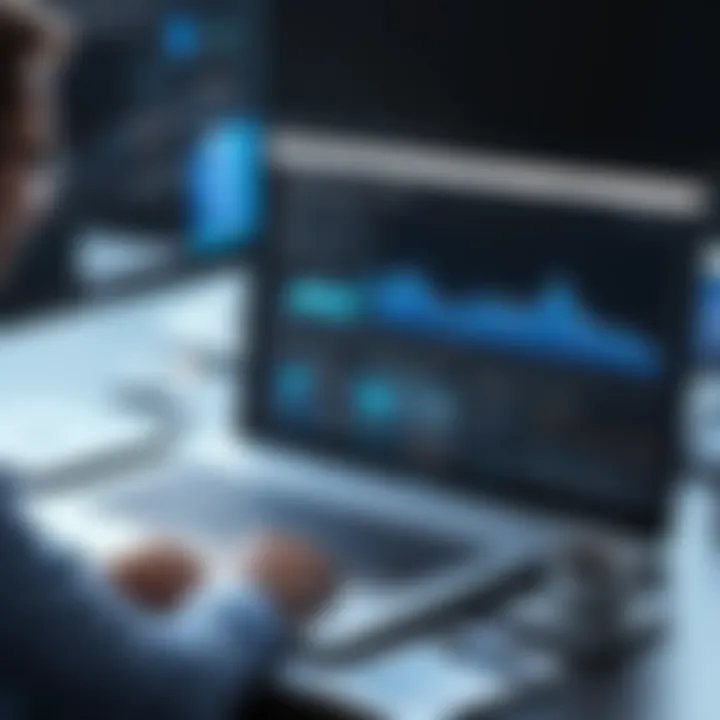
Some key benefits of integrating machine learning into statistical forecasting include:
- Enhanced Accuracy: Machine learning models can analyze complex relationships in data that might be too intricate for standard methods to capture.
- Scalability: These models can easily handle increased data volumes, whether thatās from social media trends, market fluctuations, or consumer behavior shifts.
- Automation: By automating routine forecasting tasks, businesses can save time and decrease human error.
However, it's important to keep in mind certain considerations when adopting machine learning for forecasting. Companies should ensure they have access to quality data and the technical expertise to build and maintain models; otherwise, they risk running into pitfalls such as overfitting or misinterpreting results.
"Sophisticated machine learning tools can unveil trends that often remain hidden to conventional forecasting methods."
Big Data Analytics
The emergence of big data analytics has opened new doors for statistical forecasting. Organizations now have access to large datasets that can provide insights never seen before. Data can come from a multitude of sources, including social media, sensors, web traffic, or customer interactions. The ability to tap into such extensive information can significantly improve the clarity and relevancy of forecasts.
Key aspects of how big data analytics is reshaping forecasting are:
- Real-Time Analysis: Businesses can make predictions based on the latest data, allowing them to react swiftly to market changes.
- Comprehensive Insights: Large datasets provide a more complete picture, enabling organizations to understand multifactorial relationships affecting their forecasts.
- Data Fusion: Integrating data from various sources can lead to more robust predictive models, which consider several influencing factors simultaneously.
With these benefits comes the need for careful data governance. Organizations must ensure data is collected legally and ethically to avoid compliance issues. Moreover, the presence of too much data can be overwhelming, so filtering relevant information without losing sight of key insights is essential.
In summary, the trends of machine learning integration and big data analytics are reshaping the landscape of statistical forecasting. By understanding and adapting to these innovations, businesses can position themselves to navigate the future of forecasting more effectively.
Case Studies of Successful Forecasting
Case studies play a pivotal role in demonstrating the practical application of statistical forecasting techniques. They illustrate how theoretical concepts translate into tangible results across various sectors. By dissecting real-life scenarios, businesses can glean insights that guide their own forecasting efforts, enhancing strategic planning and decision-making.
Through these detailed examinations, we can ascertain the effectiveness of different methods, appreciating not just their successes but also the challenges faced along the way. This gives organizations a realistic view of potential pitfalls and areas for improvement.
Industry Applications
Retail Sector Case Study
A prominent example in the retail sector focuses on target corporation, an entity that successfully utilized time series forecasting to optimize its inventory management. The specific aspect of this case study lies in how Target leveraged seasonal sales patterns to predict product demand ahead of peak shopping seasons. This prediction made a profound impact on inventory control, assisting in minimizing stockouts and reducing excess inventory, both of which can eat into profit margins.
The key characteristic of this study is its use of historical sales data, revealing a strong correlation between past and future sales trends. This makes it a beneficial choice as the systematic approach allows for more informed decision-making. Moreover, Targetās initiative serves as a significant example for other retailers, demonstrating that data analytics can drive results effectively.
However, the unique feature here is its adaptability to local market trends. While Targetās national data trends are crucial, the company succeeded by incorporating local events and demographics, making its forecasting even more relevant. One might consider the disadvantage being the heavy reliance on past data, which can sometimes lead to unexpected outcomes if user behavior shifts suddenly.
Healthcare Forecasting Example
In healthcare, the forecasting example of childrenās hospitals combining statistical forecasting methods with machine learning provides an enlightening perspective. Hospitals have begun applying forecasting to predict patient admission rates during flu season. This specific aspect demonstrates how they anticipate resource allocation needs, including staff and bed availability, thus ensuring better patient care.
A notable characteristic of this approach is the incorporation of real-time data, such as viral outbreak reports. This method gives hospitals the agility required to adapt forecasts as conditions evolve. It is certainly a popular choice among healthcare administrators who seek to enhance operational efficiency.
On the unique side, combining statistical models with machine learning allows hospitals to refine their forecasts continually. This iterative process presents a major advantage, as it helps catch subtle changes in data that may lead to significant fluctuations in demand. On the downside, integrating technology demands a substantial investment in training staff and upgrading systems, which might pose challenges for some institutions.
"Effective forecasting not only anticipates needs but also anticipates shifts in demand, especially in unpredictable environments such as healthcare."
Closure and Future Directions
The conclusion of this article delves into the significance of statistical forecasting in shaping future strategies for organizations. Statistical forecasting has grown beyond basic predictions, evolving into a cornerstone of strategic planning in various fields, from business to healthcare. Recognizing its far-reaching implications allows organizations to stay ahead of the curve, adapting and evolving in an ever-changing environment. As we unpack this final section, the emphasis will be on key insights garnered throughout the article and the future trajectory of statistical forecasting methods.
Summary of Key Insights
To encapsulate the insights gained from this comprehensive exploration, here are some pivotal takeaways:
- Foundational Methods: Understanding various forecasting methods, such as qualitative and quantitative approaches, enables businesses to choose suitable techniques tailored to their needs.
- Role of Data: The quality of data remains a critical factor in effective forecasting. With poor data, no method can yield reliable results.
- Technological Advances: The integration of machine learning and big data has transformed forecasting, enhancing accuracy and broadening the scope of application.
- Challenges: Despite advancements, challenges persist, particularly regarding model selection and external uncertainties that influence accuracy.
Itās clear that statistical forecasting is not merely a predictive tool; it is an essential part of the decision-making process that supports organizations in navigating uncertainty.
Evolving Landscape of Statistical Forecasting
The landscape of statistical forecasting is constantly shifting, reflecting the rapid evolution of technology and data science. Key trends are shaping its future:
- Artificial Intelligence: The adoption of AI is becoming increasingly prevalent. These systems can process vast amounts of data and identify patterns that may elude traditional methods.
- Real-Time Data: Organizations are moving towards real-time data processing, which allows for more agile decision-making. This shift offers an edge in industries like e-commerce, where consumer behavior changes rapidly.
- Collaboration Tools: As businesses become more interconnected, collaboration in data analytics and forecasting is on the rise, enabling shared insights and resource pooling.
Looking ahead, the effectiveness of statistical forecasting will likely be determined by its ability to integrate more advanced technologies and address the complexities of a data-saturated environment. The challenge remains to create methods that not only provide accurate forecasts but also adapt to future uncertainties.
"In the world of statistical forecasting, adaptability is just as crucial as accuracy."
Ultimately, as organizations forge ahead with statistical forecasting, they must remain vigilant and adaptable to harness the true potential of their data.