Exploring AI Audience Targeting in Digital Marketing
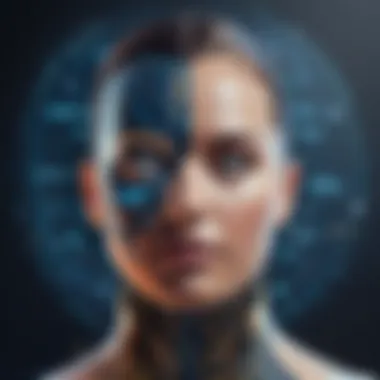
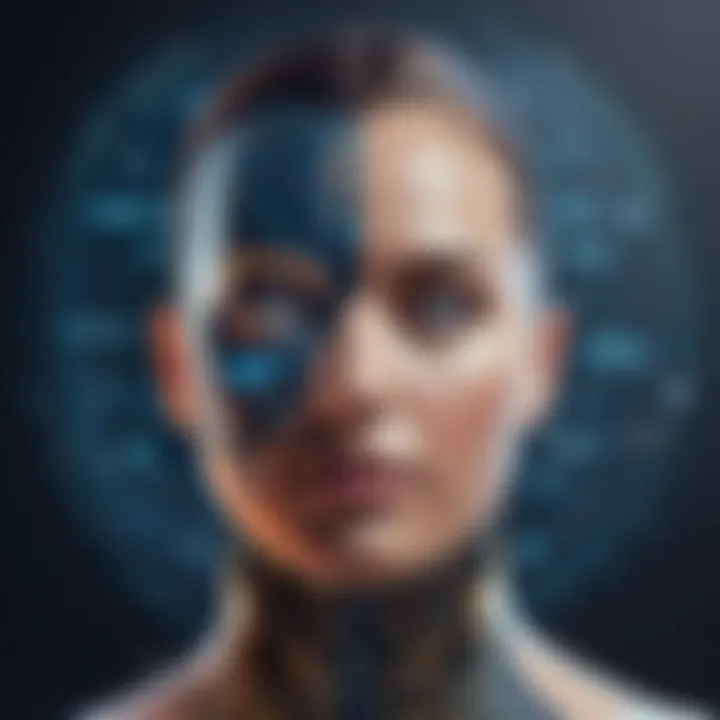
Intro
The evolution of digital marketing has profoundly transformed the way businesses engage with their audiences. It is no longer adequate to simply cast a wide net; precision is now the name of the game. Here, Artificial Intelligence (AI) emerges as a game-changer, paving the way for optimizing audience targeting strategies that are tailored, insightful, and effective.
In today's hyper-connected world, where information overload is rampant, brands must harness AI to sift through massive datasets, thereby identifying key patterns for effective communication and engagement. The ability to segment, profile, and engage audiences accurately not only saves marketing dollars but also elevates customer experiences to previously unimaginable levels.
This exploration will unravel the intricate workings behind AI-driven audience targeting, emphasizing not just the operational methodologies but also the ethical considerations and predictive capacities inherent in these systems.
Prelude to AI Audience Targeting
In today’s intricate digital landscape, companies find themselves navigating a shifting maze of consumer preferences. The ability to effectively target audiences has evolved remarkably, with AI leading the charge as the shining beacon of modern marketing. At its core, AI audience targeting leverages data analytics and machine learning to refine the intricacies of marketing strategies.
The significance of understanding AI audience targeting cannot be overstated. It offers businesses a means to not just reach, but also resonate with their intended market. The days of guesswork are fading; instead, companies now employ sophisticated algorithms that process vast amounts of data, thus allowing for refined, hyper-targeted marketing efforts.
Definition and Relevance
AI audience targeting refers to the application of artificial intelligence to identify and segment potential customers based on various parameters. This technique utilizes data-driven insights to optimize marketing initiatives, thereby increasing conversion rates and enhancing customer engagement. In essence, AI acts as a compass that points marketers in the right direction, ensuring their messages land with the audience most likely to respond positively.
The relevance of AI audience targeting is underscored by the growing importance of personalized marketing. As consumers are bombarded with an avalanche of information every day, they are likely to tune out messages that don’t speak directly to their needs or interests. A targeted approach mitigates this, paving the way for brands to foster stronger connections through tailored messaging.
"The power of AI audience targeting lies in its capacity to inform marketing decisions with pinpoint precision."
Evolution of Targeting Strategies
Understanding the evolution of targeting strategies provides valuable context for the current practices in use. In earlier times, brands relied primarily on demographic information, which often proved unsatisfactory due to its broad strokes in defining consumer behavior. As technology progressed, marketers began employing more nuanced strategies, such as behavioral targeting. This trend enabled firms to pinpoint user activity patterns, offering a more refined view of customer engagement.
Fast forward to today, and we see the tides shift once more with the integration of AI. Now, brands can analyze data in real-time and recalibrate their strategies at an astonishing rate. Whether it be through monitoring social media interactions or assessing website user behavior, AI equips marketers with tools that offer a 360-degree view of their audiences.
From the initial mass appeal tactics to the precise, data-driven methods of today, the evolution of audience targeting is a testament to the growing complexity inherent in consumer marketing. As businesses adapt to this dynamic environment, AI has emerged as an essential ingredient for successful audience targeting strategies.
Understanding the Core Concepts
Understanding the core concepts of AI audience targeting serves as a foundational block upon which effective strategies are built. Without grappling with these core ideas, marketers may find themselves groping in the dark, unable to fully harness the power of AI to connect with their desired audiences. In this section, we break down key areas, offering a clear view of how data collection and segmentation strategies intertwine to drive insightful analysis and practical action.
Data Collection Mechanisms
Data collection is the lifeblood of AI audience targeting. Without reliable data, attempts to understand consumer behavior remain largely speculative. Various mechanisms play a role in this gathering process.
- Surveys and feedback forms allow businesses to directly engage customers, posing questions about preferences and behaviors. This method provides qualitative data that can add richness to the overall analysis.
- Digital analytics tools, like Google Analytics, collect vast amounts of behavioral data anonymously. Page visits, time spent on a site, and social media interactions all serve as indicators of consumer interest. This method is often efficient and powerful, capable of drawing patterns that may be invisible through manual observation.
- Third-party data aggregators gather extensive information from various sources, combining different datasets to offer a view into consumer habits, desires, and demographic parameters. This approach helps widen the net of information, though it may raise some privacy concerns.
Leveraging diverse data collection mechanisms provides a nuanced portrait of the target audience, allowing companies to tailor their marketing efforts to specific needs and preferences.
Segmentation Techniques
Segmentation is akin to pulling out the proverbial magnifying glass to view distinct consumer groups within the broader audience. Each segment requires a different strategy, ensuring that marketing efforts resonate effectively. Here, we outline three primary segmentation techniques, their distinct characteristics, and their roles in shaping effective marketing.
Demographic Segmentation
Demographic segmentation focuses on identifiable statistics such as age, gender, income level, and education. In many ways, it's the first fork in the road for marketers when crafting audience profiles. The key characteristic here is that demographic information is relatively straightforward to obtain and analyze. This method is popular because it allows businesses to quickly target key segments without delving too deeply into the complexities of behavior or preference.
One unique feature of demographic segmentation is its ability to create profiles based on broad characteristics, making it easy to accommodate large volumes of potential customers. However, it can sometimes oversimplify the audience, leaving out nuanced behavioral or psychological factors that might drive engagement. Thus, while useful, it shouldn’t be the sole strategy employed.
Behavioral Segmentation
Behavioral segmentation dives deeper than demographics by analysing user actions, such as purchasing patterns, browsing habits, and feedback responses. What sets this method apart is its reliance on actual user behavior rather than assumptions based on age or gender alone. This makes it a beneficial choice for many marketers today.
The unique aspect of behavioral segmentation is its adaptability. Businesses can pivot strategies in real time based on observed behaviors, catching the wave of interest before it wanes. However, analyzing behavior can be complex, requiring sophisticated tools and expertise to derive insights accurately.
Psychographic Segmentation
Psychographic segmentation goes beyond what users do to explore why they do it—considering interests, values, and lifestyle choices. This technique brings a richer layer of understanding to audience profiling.
The standout characteristic of psychographic segmentation is its focus on emotional and psychological triggers. It enables marketers to connect with audiences on a more profound level, enhancing engagement through tailored messages that resonate deeply. However, the challenge lies in gathering and interpreting psychographic data, which can require more in-depth research and understanding.
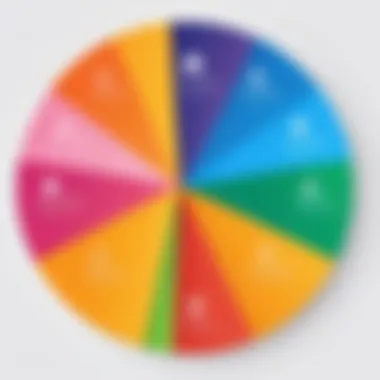
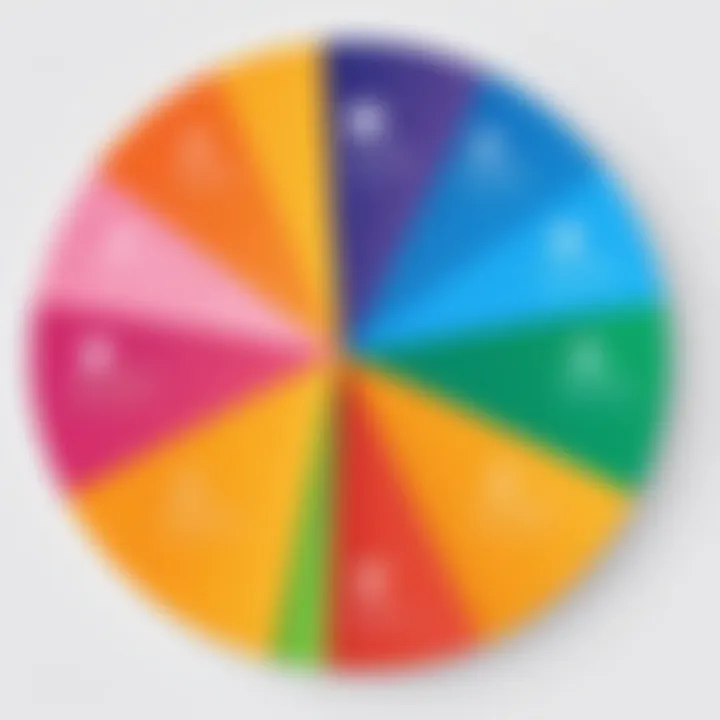
In summary, understanding the core concepts of data collection and segmentation techniques allows businesses to create more effective, targeted marketing strategies that resonate with diverse audiences. Knowledge of these mechanisms is paramount to navigating the evolving landscape of AI audience targeting.
AI Technologies in Audience Targeting
AI technologies have become essential tools in audience targeting for several reasons. It allows marketers to analyze vast amounts of data quickly and make real-time decisions. With the increasing complexity of consumer behavior, harnessing AI gives businesses a competitive edge. By utilizing powerful algorithms, they can enhance engagement and improve conversion rates effectively.
Machine Learning Algorithms
Supervised Learning
Supervised learning's primary strength lies in its ability to learn from labeled datasets, allowing for predictions based on historical data. This aspect is particularly important in audience targeting, where identifying patterns can inform marketing strategies. A key characteristic of supervised learning is its reliance on training data to create a model that predicts outcomes or classifies information. This method has gained popularity due to its effectiveness in tasks like email categorization or content recommendations.
One unique feature of supervised learning is its ability to improve accuracy over time. As more data becomes available, the models can be refined, which directly benefits the strategies executed in audience targeting. However, it does have its disadvantages. Requires a substantial amount of labeled data, which can be a barrier for some businesses.
Unsupervised Learning
On the flip side, unsupervised learning tackles datasets without pre-existing labels. This facet makes it invaluable for discovering hidden patterns or groupings within data, particularly in audience segmentation. The ability to analyze data points without predefined notions allows for greater flexibility and creativity in targeting strategies. A notable characteristic of unsupervised learning is how it clusters similar groups based on behavior or preferences, often presenting unexpected consumer insights.
This unique capability enables businesses to adjust their approaches based on new trends identified through data analysis. While beneficial, unsupervised learning does have its drawbacks. The lack of clear objectives can sometimes lead to ambiguity in results, making interpretation challenging.
Natural Language Processing
Natural Language Processing (NLP) enhances audience targeting by enabling machines to understand human language nuances. Marketers today use NLP alongside machine learning algorithms to analyze customer sentiment across various platforms. This allows for adjustments in messaging based on real feedback from consumers. Through analyzing reviews, social media interactions, or user comments, NLP provides insights into how audiences perceive a brand or product.
Moreover, NLP facilitates personalization on a higher level. Conversations can be tailored based on language use and preferences, creating a more engaging user experience.
Overall, the integration of AI technologies like machine learning and NLP allows businesses not just to target audiences more effectively but also to foster a deeper understanding of consumer behaviors, ultimately driving better results.
In the evolving landscape of digital marketing, AI technologies are not just tools; they are the backbone of effective audience targeting strategies.
By embracing these innovations, companies can navigate the complexities of modern consumer behavior more adeptly and make informed decisions that resonate with their target audiences.
Personalization Strategies Utilizing AI
When it comes to digital marketing, personalization takes the cake. In a world brimming with data, AI helps sift through mountains of information to deliver tailored content. This ensures that consumers aren't just another face in the crowd; they resonate with brands on a more personal level. By leveraging AI in personalization strategies, businesses can engage their audience in meaningful ways.
Dynamic Content Generation
Dynamic content generation is akin to having a tailor on hand to craft bespoke suits, except we're crafting digital experiences in real-time. This strategy involves AI algorithms adjusting web content based on user interactions, preferences, and behavior. For instance, if a user consistently browses eco-friendly products, the AI might showcase green alternatives on their next visit, making the browsing experience feel more intuitive.
The benefits are manifold:
- Increased Engagement: When users see content that aligns with their interests, they're more likely to interact, share, and make purchases.
- Enhanced Customer Experience: A personalized touch can make users feel valued, increasing their loyalty to the brand.
- Higher Conversion Rates: Tailored content often translates to better conversion rates, giving companies a more substantial return on their investment.
However, businesses must tread carefully here. While dynamic content can resonate with audiences, overstepping boundaries can lead to misguided privacy concerns. Data collection methods must be transparent and ethical, or businesses risk losing user trust.
"The ability to tailor experiences is no longer a luxury; it's a necessity in a data-driven world."
Predictive Analytics
Predictive analytics utilizes historical data and AI techniques to forecast future user behavior. Imagine peering into a crystal ball that informs companies of which products will attract customers next season, based on past behaviors. This is where AI shines; it turns numbers into insights, aiding brands in strategic decision-making.
This process involves several steps:
- Data Collection: Gathering various data points, from purchase history to browsing patterns.
- Data Analysis: Using machine learning algorithms to identify trends and patterns.
- Prediction: Generating forecasts on future behavior, be it likely purchases or product interest.
The advantages here cannot be understated:
- Targeted Marketing Campaigns: With predictions in hand, brands can fine-tune their marketing efforts, aiming at right audience segments with precision.
- Resource Optimization: Predictive analytics allows brands to allocate resources efficiently, targeting markets that show the highest potential.
- Risk Management: Predicting consumer behavior also helps in alleviating risks, ensuring businesses are a step ahead of market trends.
In sum, personalization strategies utilizing AI are revolutionizing the marketing landscape, supplying companies with the tools to foster authentic connections. The dynamic interplay between content generation and predictive analytics not only enhances the customer experience but also drives the bottom line. As we move forward, adapting these strategies will distinguish the leaders from the laggards in this competitive arena.
Ethical Considerations in Audience Targeting
The landscape of AI audience targeting isn’t just about enhancing marketing efficiency; it’s also about navigating the murky waters of ethics that accompany such sophisticated technologies. In this rapidly changing digital world, it’s essential to approach audience targeting with a sense of responsibility and transparency. This section delves deep into the ethical implications surrounding audience targeting, focusing on two critical areas: data privacy concerns and biases inherent in algorithms.
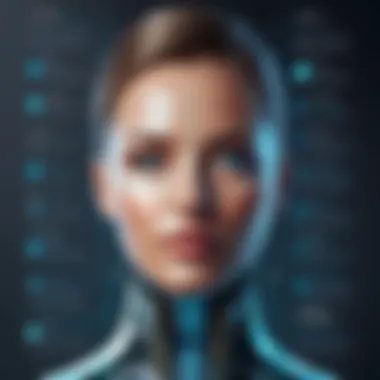
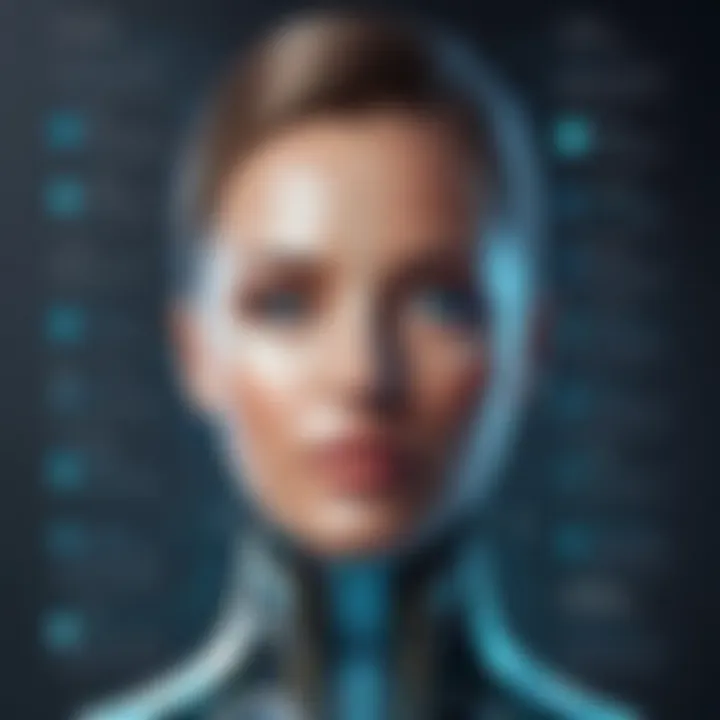
Data Privacy Concerns
At the heart of ethical considerations lies data privacy. In an era where personal information is often referred to as the "new oil," safeguarding it becomes not just a priority but a moral obligation. The collection, use, and potential misuse of consumer data has prompted heated debates and regulations such as the General Data Protection Regulation (GDPR) in Europe.
Collecting data is one piece of the puzzle, but how it's utilized is paramount. For instance:
- Informed Consent: Consumers must be educated about how their data will be used. They should be enabled to provide explicit consent. If marketing practices feel misleading, trust evaporates.
- Transparency: Brands have a responsibility to clarify their data practices. When a customer feels they are being manipulated, the relationship can turn sour. Clear communication helps foster authenticity.
- Data Minimization: Organizations should collect only the data necessary to achieve a specific purpose. Excessive data collection can lead to breaches and increased risks for consumer trust.
The ethical use of data isn't just good practice; it can also turn customer relations from transactional to relational. When consumers feel secure, they are more likely to engage with brands that respect their privacy. This kind of connection does wonders for brand loyalty.
"Privacy is not an option, and it shouldn't be the price we accept for just getting on the internet. We deserve better. We deserve a web that respects our privacy." — @questions on reddit
Bias in Algorithms
Algorithms are often perceived as infallible machines. However, they are only as unbiased as the data that trains them. This leads to the second critical aspect of ethical considerations: biases in algorithms. If the data fed into an algorithm reflects existing stereotypes or neglects certain demographics, the results produced can perpetuate inequality.
Several factors contribute to algorithmic bias:
- Historical Data: Training data can encapsulate past prejudices. For example, in employment data, if biases existed against particular groups, those biases can continue to play out in hiring algorithms, disadvantaging qualified individuals.
- Lack of Diversity in Development Teams: If the teams creating these algorithms lack diverse viewpoints, they may inadvertently embed their blind spots into the algorithms. A homogeneous team might overlook how certain decisions impact varied audiences.
- Feedback Loops: Algorithms that learn from ongoing interactions may inadvertently reinforce biases. For instance, a recommendation algorithm that prioritizes popular choices may disregard niche audiences, perpetuating a cycle of exclusion.
Addressing bias requires active intervention, including:
- Regular Audits: Routine evaluations of algorithms can help identify and rectify biases before they cause harm.
- Inclusive Datasets: Seeking diverse data sources ensures that algorithms reflect a broader spectrum of experience and opinion.
- Feedback Mechanisms: Engaging end users in the evaluation process can highlight unnoticed biases and improve overall accuracy.
Ultimately, addressing these ethical concerns isn't merely a checkbox exercise. It’s about commitment to responsible practices that uphold consumer rights and enhance the trust that fuels the digital ecosystem. In this rapidly evolving field of AI audience targeting, the ability to balance effective marketing with ethical considerations will likely shape the future trajectories of businesses everywhere.
Challenges in AI Audience Targeting
In today’s fast-paced digital marketing arena, leveraging AI for audience targeting is a double-edged sword. While the technology holds promising potential, it does not come without its skein of challenges. Recognizing these hurdles—ranging from the integrity of data to the fluid nature of consumer behavior—is crucial for marketers who aim to utilize AI effectively. This section delves into these challenges, providing a nuanced view that highlights their implications on strategy and execution.
Data Quality Issues
The bedrock of AI audience targeting lies in the veracity of data. When data quality is compromised—whether through inaccuracies, biases, or incompleteness—the results can be misleading. Poor data quality can lead to irrelevance in targeting; think of a personalized ad directed at a demographic that has no interest in the product. This scenario not only wastes resources but risks alienating potential customers.
Key Aspects of Data Quality
- Accuracy: Is the data correct? If your analytics rely on flawed information, your audience insights are set on shaky ground.
- Completeness: Having partial information regarding user behavior or preferences can skew results.
- Timeliness: Data should be up to date. In the world of marketing, yesterday’s insights can quickly become today’s relics.
The ramifications of these issues extend to algorithmic training. A machine learning model trained on subpar data may perpetuate existing biases, exacerbating marketing inefficiencies. It’s imperative that businesses not only collect vast amounts of data but ensure its quality through rigorous validation processes.
"Quality data is like gold; it's the basis of effective marketing strategies. Without it, efforts can go down the drain quickly."
Evolving Consumer Behaviors
Another pivotal challenge is grappling with the ever-changing landscape of consumer behavior. Today, consumers are bombarded with an incessant stream of information and advertising, shaping their expectations and reactions continuously. As new trends emerge, consumer preferences can shift rapidly, sometimes overnight.
Factors Influencing Consumer Behavior
- Technological Advancements: The integration of new technologies (like augmented reality and chatbots) alters how consumers engage with brands.
- Social Influences: Social media trends can make or break a marketing campaign almost instantaneously, requiring AI systems to adapt on the fly.
- Cultural Shifts: With changes in societal norms and priorities, brands must remain sensitive and responsive to these shifts.
For instance, a generational shift toward sustainability can render traditional marketing strategies obsolete. Brands that recognize and adapt to these changes can improve engagement and enhance customer loyalty, whereas those that do not risk becoming irrelevant. This dynamic nature of consumer behavior underscores the necessity of continuous data collection and analysis to refine audience targeting strategies.
In summation, while AI opens doors for more refined audience targeting, marketers must confront the labyrinth of data quality issues and evolving consumer behaviors. Understanding and navigating these challenges is essential to harnessing the true potential of AI in audience targeting.
Real-World Applications of AI Targeting
Understanding how AI audience targeting operates in real-world settings is crucial for grasping its significance in various industries. The applications of AI-driven targeting are vast and interdisciplinary, helping businesses tailor their approaches to best suit the needs of their specific audience. With the right strategies, companies can harness this technology to enhance customer experiences, boost sales, and improve brand loyalty.
One of the standout elements of AI targeting is its ability to deliver personalized and relevant content directly to consumers. This is not just about putting products in front of potential buyers; it’s about crafting experiences that resonate. As consumers become more discerning, the ability to meet them with precisely what they're looking for—often before they even realize they want it—is what setting brands apart. The benefits are numerous:
- Increased engagement rates through tailored marketing efforts.
- Improved customer satisfaction, as messages feel more relevant.
- Higher conversion rates stemming from timely and pertinent offers.
However, leveraging these applications doesn't come without considerations. Companies must strike a balance between leveraging data and ensuring customer privacy. Trust, once broken, is hard to rebuild. Ethical practices in data management are paramount; transparency with consumers about how their data is used is not just a good idea, but a necessity.
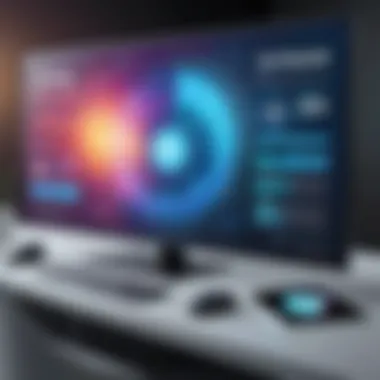
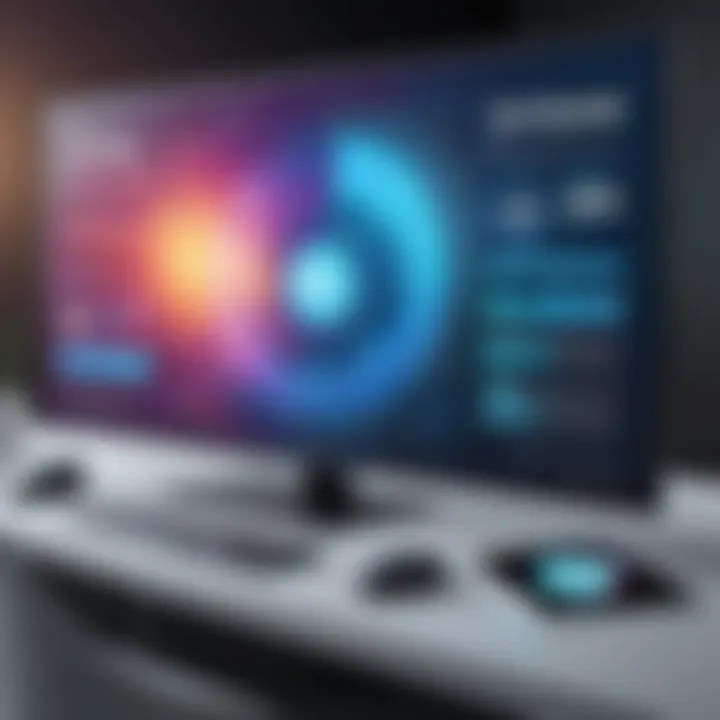
Case Studies in E-commerce
In the e-commerce landscape, AI targeting has become the backbone of a successful marketing strategy. Companies like Amazon have excelled through personalized recommendations. When you visit their website, it is not just a store; it's a tailored shopping experience based on user behavior and preferences.
AI systems analyze massive datasets, including past purchases, search queries, and even items left in shopping carts, to suggest products uniquely suited to each shopper. This process not only enhances the user experience but also significantly increases sales. Reports indicate that personalized recommendations account for about 35% of Amazon’s total revenue.
"In today’s e-commerce market, standing still is akin to moving backward. AI is the fuel that drives innovation and enhances user experience."
Other e-commerce giants like eBay andAlibaba have adopted similar approaches. eBay's use of AI to automate customer service inquiries has led to faster response times and increased customer satisfaction. Moreover, Alibaba employs AI to optimize pricing in real-time, ensuring competitive pricing without human intervention. As the market evolves, these case studies illustrate that when it comes to e-commerce, AI-driven audience targeting isn’t just a luxury; it’s a necessity.
Applications in Social Media Marketing
On social platforms, AI targeting is reshaping how brands connect with users. The ability to create highly targeted ad campaigns is transforming social media marketing into something far more effective. For instance, Facebook’s advertising platform leverages AI to analyze user data, enabling businesses to reach their ideal clients with precision.
Marketers can choose specific demographics, interests, and even behaviors to identify the most receptive audience for their campaigns. Successful brands are now running ads that speak directly to niche audiences; for example, a company might target eco-conscious consumers with ads for sustainable products, taking into account their online behaviors and preferences.
Furthermore, platforms like Instagram and Snapchat are utilizing augmented reality to present immersive ads calibrated by AI analytics. The results? Users are not just seeing ads—they’re experiencing them, creating deeper emotional connections with the brand.
In summary, the real-world applications of AI targeting in e-commerce and social media not only enhance customer engagement but redefine the potential of digital marketing strategies. Brands that navigate these waters wisely will likely find themselves at the forefront of innovation and customer satisfaction.
Future Trends in AI Audience Targeting
In the fast-paced realm of digital marketing, grasping the future trends in AI audience targeting becomes not just relevant but essential. The landscape is shifting rapidly, driven by technological advancements and evolving consumer behaviors. Understanding these changes ensures that businesses can adapt their strategies, remain competitive, and effectively engage with their audience.
Integration with IoT Devices
The integration of Artificial Intelligence with Internet of Things (IoT) devices signifies a crucial step in refining audience targeting. IoT devices – from smart homes to wearable technology – continually generate vast amounts of data. This data can provide marketers with an unprecedented level of insight into consumer behavior and preferences.
For instance, imagine a smart thermostat collecting data on a user’s heating preferences. If this data is mined effectively, businesses can tailor their marketing strategies to better align with the homeowner’s habits, leading to more personalized advertising. With the ability to analyze when a user is most likely home, based on temperature adjustments, companies can serve ads during those peak times, increasing the chances of engagement.
Moreover, integrating AI with IoT can offer predictive capabilities, allowing marketers not just to react to consumer preferences but to anticipate them.
- Benefits of IoT Integration:
- Enhanced consumer insights
- Timely and relevant marketing campaigns
- Improved customer experience
This level of integration also raises important considerations concerning privacy. As devices gather extensive amounts of sensitive data, ensuring that this data is handled ethically and securely will be paramount.
Advancements in AI Technologies
As AI technologies evolve, their impact on audience targeting becomes more profound. Recent advancements in neural networks, deep learning, and natural language processing play a pivotal role in enabling marketers to derive deeper insights and accuracy in their targeting.
- Deep Learning: This subset of machine learning allows for more complex data analyses by simulating the functioning of the human brain in understanding patterns. Marketers can leverage deep learning to analyze unstructured data like social media posts and customer reviews, deriving nuanced understanding of consumer sentiment.
- Natural Language Processing: With enhanced NLP capabilities, AI can decipher context, slang, and even sentiment in customer interactions. For example, if a consumer tweets about dissatisfaction with a product, businesses can respond rapidly, potentially restoring customer relations before they escalate.
- Predictive Analytics: Utilizing these advanced technologies, companies can create highly sophisticated models that not only track current audience behaviors but also predict future actions. This enables proactive strategies rather than reactive ones, which is a game changer in maintaining relevance and engagement.
"Advancements in AI technologies equip marketers with tools that not only enhance efficiency but also drastically improve the quality of insights provided about the target audience."
The combination of these technologies fosters a more agile marketing approach, where teams can adjust campaigns in real-time based on instantaneous consumer feedback.
In summary, as we look towards the future of AI audience targeting, the integration with IoT devices and advancements in AI technologies stand out as not just trends, but necessities for effective audience engagement. Understanding these elements will arm marketers with the tools needed to create personalized, timely, and relevant interactions, ensuring they remain at the forefront of their industries.
Culmination
In wrapping up the exploration of AI audience targeting, it’s crucial to underline the significance this topic holds in the digital marketing landscape today. The overarching message is clear: to navigate the evolving marketplace effectively, understanding the mechanisms of AI-driven strategies is not optional but essential. As we delved into various facets of audience targeting, several key elements emerged as paramount.
Synthesizing Insights
Understanding AI audience targeting goes beyond mere implementation of technology—it’s about harnessing data to craft tailored experiences for consumers. This article dissected numerous insights, emphasizing that effective audience targeting is rooted in:
- Data Quality: Not all data is created equal. Ensuring accuracy and reliability is foundational. Poor data leads to misguided strategies that can tarnish a brand's reputation.
- Consumer Behavior: Staying attuned to the shifts in consumer preferences and trends is critical. Targeting strategies must be adaptable, responding promptly to the ever-changing tides of market needs.
- Ethical Considerations: As mentioned in earlier sections, the ethical implications surrounding data privacy and algorithmic bias cannot be overlooked. A company's transparency in utilizing audience data can become a competitive advantage.
In synthesizing these insights, one must appreciate how intertwining technology with consumer understanding not only enhances targeting precision but also fosters trust, an invaluable currency in today's market.
Actionable Recommendations
Based on the analysis provided throughout the article, there are several actionable steps that professionals can take to refine their audience targeting strategies:
- Invest in Data Management Solutions: Utilize robust data management platforms to enhance the quality and integrity of collected data. This positions businesses to derive actionable insights reliably.
- Adopt Advanced Segmentation Techniques: Move beyond traditional demographic segmentation to incorporate behavioral and psychographic techniques.
- Regularly Audit Your Algorithms: With the rise of AI, algorithms have substantial influence. Conduct periodic audits to ensure they are free from biases, reflecting your commitment to ethical practices.
- Stay Updated on Regulations: Familiarize yourself with data privacy regulations that may affect your targeting strategies. Compliance should be integrated into your operational protocols to minimize risks.
- Leverage Predictive Analytics: Use tools that allow for predictive analytics to anticipate consumer needs and preferences. Being proactive rather than reactive can significantly enhance engagement rates.
- Use tools that allow for dynamic segmentation based on real-time data feeds.
- This enables a more personalized experience for each user, ultimately increasing engagement.
By adopting these recommendations, businesses can position themselves at the forefront of AI audience targeting, equipped with strategies that not only engage but also foster profound consumer relationships. The rapidly changing digital landscape demands such agility, and those who successfully adapt will reap the rewards of informed, data-driven decisions.