Snowflake Database Competitors: In-Depth Analysis
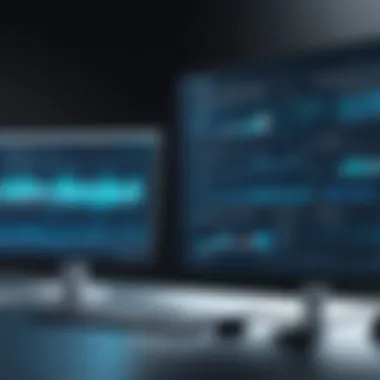
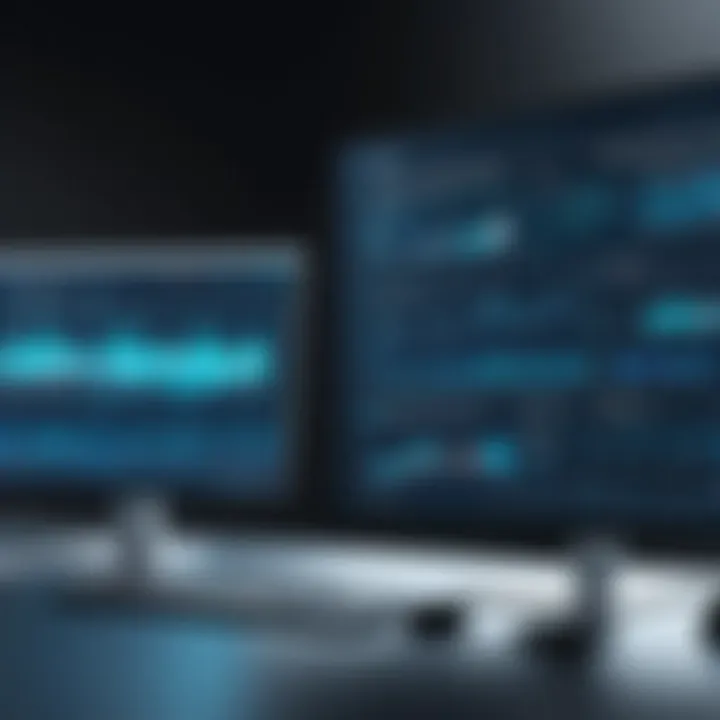
Intro
The landscape of data management is ever-evolving. As organizations strive to enhance their analytical capabilities, choosing the right database solution becomes critical. The Snowflake database has emerged as a strong contender in this arena, but it is essential to explore its competitors. Doing so provides valuable insights into alternative solutions that might better suit specific organizational needs.
In this analysis, we aim to delve into the functionalities and scalability of competing databases. This will help business leaders and IT specialists make informed choices. Our examination will focus on features, performance metrics, and user experiences. By dissecting the strengths and weaknesses of these alternatives, we hope to present a clearer view of what the market offers.
Understanding these aspects can significantly impact decision-making processes. The right choice of database can enhance operational efficiency, streamline analytics, and support growth. Therefore, this article will serve as a guide, detailing key points for evaluating competitors of Snowflake.
Prolusion to Snowflake
Snowflake has emerged as a key player in the realm of cloud data warehousing, reshaping how businesses handle and analyze their data. Understanding Snowflake's architecture and features is crucial for making informed decisions about data storage and analytics solutions. In this article, we will explore various competitors to Snowflake, evaluating their strengths and shortcomings. This analysis helps illuminate the choices before organizations navigating the complex landscape of data solutions.
Snowflake distinguishes itself through its unique architecture that facilitates scalability and performance. By diving into the specifics of Snowflake, we equip technical professionals and business leaders with insights critical for selecting the right tools for data management and analysis.
The relevance of focusing on Snowflake lies in its transformative impact on data handling processes. As companies generate vast amounts of data, the demand for efficient storage and retrieval mechanisms has become paramount. Snowflake provides a compelling answer, but it is not the only solution available.
Overview of Snowflake Architecture
Snowflake operates on a multi-cloud architecture, which allows users to deploy the platform across different cloud providers. This structure provides flexibility, enabling organizations to choose their preferred cloud environments such as Amazon Web Services, Microsoft Azure, or Google Cloud Platform. The architecture separates compute from storage, which means that users can scale each independently based on their needs. This separation not only optimizes performance in data processing but also enhances cost-effectiveness for users.
A key component of Snowflake's architecture is its virtual warehouses. These warehouse clusters manage compute resources efficiently and allow for concurrent querying without compromising performance. Each virtual warehouse can operate independently, enabling multiple teams to work simultaneously on different projects without performance degradation.
Key Features of Snowflake
Snowflake is endowed with several features that cater to the demands of modern enterprises. Among these features are:
- Automatic Scaling: Snowflake can automatically adjust compute resources based on workload demands, ensuring optimal performance without manual intervention.
- Time Travel: This functionality allows users to access historical data for a specified period, giving businesses the ability to track changes and recover previous data states.
- Secure Data Sharing: Snowflake enables organizations to securely share data in real-time with stakeholders, partners, or teams, improving collaboration while maintaining data security.
- Data Cloning: Users can create clones of data without physically duplicating it, thus saving storage costs and allowing for fast testing and development.
In summary, understanding Snowflake's architecture and capabilities is essential for evaluating its potential against competitors. The next sections will unpack the competitive landscape, examining various databases that challenge Snowflake's position in the market.
Understanding the Competitive Landscape
In the rapidly evolving arena of data warehousing, understanding the competitive landscape is essential. This knowledge enables organizations to make informed decisions about which database solution best aligns with their needs. Each platform offers distinct capabilities, price points, and features that cater to various business requirements. By analyzing competitors, businesses can better anticipate their own needs and the trends that could influence their choice of database technology.
Market Trends in Cloud Data Warehousing
Current market trends demonstrate a significant shift towards more scalable, flexible, and efficient cloud data warehousing solutions. Companies prioritize integration of advanced analytical capabilities and real-time data processing to remain competitive. Furthermore, data security and compliance have become paramount as businesses transition to cloud environments.
Some key trends include:
- Increased adoption of serverless architectures: These structures allow for automatic scaling and reduced infrastructure management, freeing up resources for other tasks.
- Focus on cost efficiency: As competition intensifies, providers are encouraged to offer more flexible pricing models, contributing to more competitive market dynamics.
- AI and ML integration: Businesses want insights from data, and machine learning algorithms help in predictive analytics, further driving the demand for sophisticated data solutions.
These trends reflect a broader push towards harnessing data capabilities in a way that aligns with business goals.
Key Players in the Market
The landscape of cloud data warehousing is populated with several formidable competitors. Each has carved a niche based on its unique offerings, performance metrics, and pricing strategies. Notable players include:
- Amazon Redshift: Known for its robust performance and deep integration with AWS services. Redshift remains a popular choice for organizations already within the Amazon ecosystem.
- Google BigQuery: Distinguished by its ability to handle massive datasets efficiently with a focus on real-time analytics. It is favored by businesses requiring quick insights from their data.
- Microsoft Azure Synapse Analytics: Offers extensive integration with Microsoft products, making it a strong candidate for enterprises utilizing Microsoft tools and services.
- Oracle Autonomous Database: This solution emphasizes automation and self-repair capabilities, seeking to reduce operational overhead for users.
- IBM Db2 Warehouse: Known for its advanced analytics and data governance features, appealing to organizations with stringent compliance needs.
- Teradata Vantage: Targets large enterprises needing performance at scale, with support for complex analytic workloads.
By knowing these key players, companies can analyze their options against specific requirements, helping in the decision-making process.
"Understanding competitors informs choices and empowers businesses to select solutions that align with their objectives."
In summary, understanding the competitive landscape serves as a foundational aspect of selecting the right database solution. By acknowledging market trends and identifying key players, organizations are better equipped to navigate their decisions in a crowded marketplace.
Amazon Redshift as a Competitor
Amazon Redshift has emerged as a significant player in the cloud data warehousing space. Recognizing its capabilities in handling large datasets efficiently, many organizations consider it a key competitor to Snowflake. This section explores various facets of Amazon Redshift, emphasizing architecture, performance metrics, cost, and user experiences.
Architecture and Scalability
Amazon Redshift employs a columnar storage architecture, which is advantageous for performing analytical queries quickly. Data is stored in a compressed format, resulting in reduced disk space utilization and faster query performance. It uses a massively parallel processing (MPP) architecture. This allows it to scale by adding more nodes to accommodate growing data volumes.
Furthermore, Amazon Redshift offers two deployment options: a single node for smaller workloads and a multi-node configuration for larger applications. This flexibility is crucial for businesses that anticipate fluctuating data demands.
Performance Metrics
Performance is a critical metric when evaluating data warehousing solutions. Amazon Redshift claims providing exceptional performance through advanced features like result caching, optimized query execution, and workload management. According to several benchmarks, its performance can be comparable to that of traditional on-premises data warehouses. However, it is essential for users to monitor and optimize their clusters for the best performance.
"Every delay in report generation translates into lost opportunities for decision-making."
Cost Considerations
When examining cost, Amazon Redshift operates on a pay-as-you-go model. Pricing is mainly based on the resources utilized, such as storage and compute nodes, which can be appealing for startups and smaller organizations. However, costs can escalate with the requirement for additional nodes or if there is excess data to store. It is crucial for businesses to carefully evaluate their consumption patterns to avoid unexpected expenses. Additionally, Amazon offers Reserved Instance pricing for companies willing to commit to longer terms, potentially yielding significant savings.
User Experiences
User reviews of Amazon Redshift vary, often shedding light on specific experiences. Many users appreciate the integration with other AWS services, enhancing the end-to-end data pipeline. On the downside, some users mention challenges related to querying complex datasets and the learning curve for optimizing the architecture. Generally, consumer satisfaction varies between organizations; thus, it is essential for potential users to consider their unique needs before opting for this platform.
Google BigQuery’s Position in the Market
Google BigQuery has rapidly established itself as a leading cloud-based data warehousing solution. Its significance in this analysis cannot be understated, as it presents a formidable alternative to Snowflake. The vast array of functionalities and its integration capabilities uniquely position it in the market. Businesses that are heavily invested in Google Cloud ecosystem find BigQuery especially appealing due to its seamless operation with other Google services and innovative features.
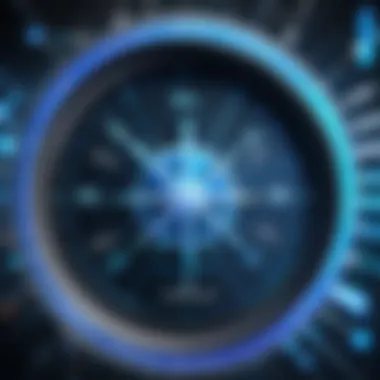
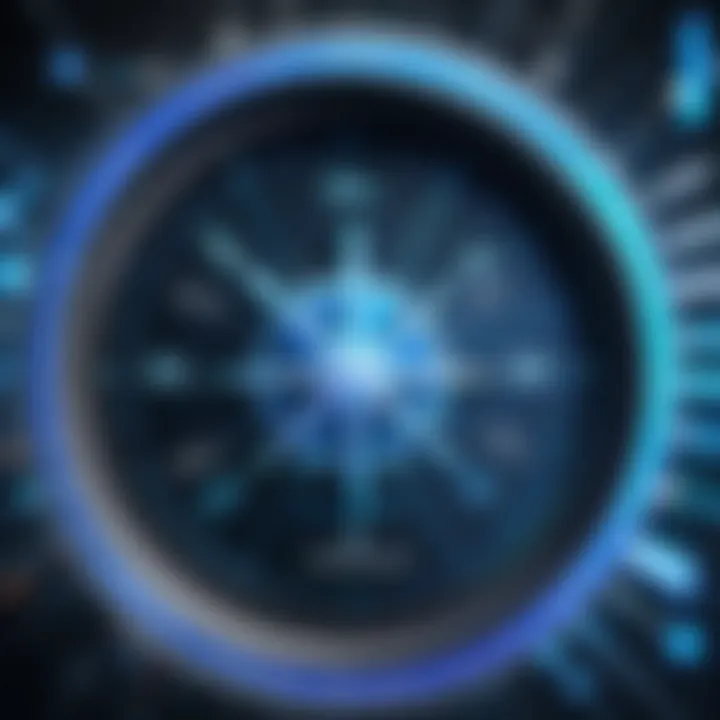
Unique Features of BigQuery
BigQuery stands out for several unique features that enhance its usability. First, it operates on a serverless architecture. This means users do not need to manage the infrastructure, which can reduce operational overhead. Moreover, its capacity for handling large datasets is impressive. BigQuery can process terabytes of data at remarkable speeds, thanks to its parallel processing capabilities.
Another notable aspect is its support for SQL queries, making it accessible for teams already familiar with SQL. This feature eliminates steep learning curves, easing the transition for many organizations. Additionally, BigQuery includes built-in machine learning capabilities, simplifying advanced analytics within the same platform. With these features, organizations can maximize their data utilization without excessive resource allocation.
Integration with Google Services
Integration with existing tools is a critical aspect for many enterprises. Google BigQuery shines in this area, particularly for organizations leveraging Google Workspace. The service integrates smoothly with tools like Google Sheets, Google Data Studio, and Google Cloud Storage. Users can easily import data from these applications with simple drag-and-drop functionality.
Furthermore, BigQuery’s compatibility with other Google Cloud services, such as Google Kubernetes Engine, enhances its value for businesses that rely on multiple services within the Google ecosystem. This integration facilitates a cohesive environment for data management, analysis, and application deployment.
Pricing Model Analysis
Understanding the pricing structure is essential for any business considering BigQuery. BigQuery employs a unique on-demand pricing model that charges users based on the amount of data processed by queries. There is also a flat-rate option available, allowing businesses to pay a set fee for increased predictability in budgeting. This flexibility accommodates various needs, whether for sporadic usage or continuous data analysis.
However, companies must be cautious of costs accumulating from querying large datasets frequently. It's essential to monitor and optimize queries to manage expenses effectively. This pricing model offers both advantages and pitfalls, making it critical for organizations to assess their specific usage scenarios thoroughly.
Customer Feedback and Case Studies
Customer feedback reveals an overall positive reception of Google BigQuery. Many users have praised its speed and ease of use. One common sentiment is that it significantly reduces time spent on data processing and analysis, allowing teams to focus on deriving insights rather than managing the complexities of data storage.
Case studies illustrate the impact of BigQuery on diverse industries. For instance, a retail company reported improved sales forecasting by utilizing BigQuery's machine learning features to analyze historical sales data swiftly. Another example includes a healthcare organization which streamlined its data collection processes, ultimately enhancing patient outcomes.
"BigQuery transformed our approach to data analysis. Its speed and integration capabilities are unmatched in our experience."
- A satisfied Google BigQuery user
Overall, Google BigQuery’s unique features, strong integration with Google services, adaptable pricing model, and positive customer reviews position it as a prominent player in the current cloud data warehousing landscape.
Microsoft Azure Synapse Analytics: Capabilities and Limitations
Microsoft Azure Synapse Analytics stands as a prominent figure in the cloud data warehousing space. Its capabilities and limitations are essential to understand for businesses considering a snowflake database alternative. The service provides a unified experience that combines big data and data warehousing, which can be highly beneficial for organizations that require versatile data management solutions.
Integration with Microsoft Ecosystem
One of the key strengths of Azure Synapse Analytics is its seamless integration with the Microsoft ecosystem. Organizations that are already using other Microsoft products, such as Office 365, Dynamics 365, or Power BI, will find it easy to incorporate Synapse into their existing workflows. The platform allows for direct data access from various sources within the ecosystem, making it easier to build analytics applications and dashboards.
Furthermore, it supports numerous data sources, including Azure Blob Storage, Azure Data Lake Storage, and SQL databases. Users can easily leverage existing Microsoft tools to enhance their data analytics capabilities, promoting streamlined processes and improved collaboration across teams. This integration fosters a cohesive environment that enhances productivity and allows for greater insight extraction from data.
Performance Evaluation and Scalability
When evaluating performance, Azure Synapse Analytics shines in its ability to scale resources based on demand. The architecture supports both on-demand and provisioned query models, giving users flexibility in managing costs while ensuring performance demands are met. For organizations that deal with varying workloads, this means they can adjust resources dynamically, minimizing expenses during low-usage periods and scaling up during peak times.
Additionally, Synapse’s use of massively parallel processing enables it to handle large volumes of data efficiently. This technology is crucial for real-time analytics, allowing businesses to derive insights quickly. However, it’s worth noting that some users have reported latency issues during peak usage periods, which can impact performance. Balancing resource allocation during high-demand times is crucial for optimal efficiency.
User Cost Analysis
Cost is always a significant factor in database selection, and Azure Synapse Analytics offers multiple pricing models to accommodate different business needs. Organizations can choose between pay-per-query for on-demand workloads or committed use for provisioned resources. This allows for flexibility and can lead to cost savings if managed wisely.
However, users should be aware of potential hidden costs. For example, data movement and integration processes can incur additional charges, which can add up quickly if not monitored carefully. Organizations must conduct a detailed cost analysis to understand all potential expenses associated with using Synapse Analytics.
Insights from User Reviews
User feedback on Azure Synapse Analytics is mixed but generally positive. Many users appreciate the platform’s robust features and its capacity to process large datasets efficiently. For businesses already within the Microsoft ecosystem, integration and usability receive high marks.
However, there have been criticisms regarding the learning curve associated with the platform. New users may find it challenging to navigate the extensive features and capabilities effectively. Some users also mention the need for improved documentation and support resources.
In summary, while Azure Synapse Analytics offers powerful capabilities and rich integration possibilities, businesses must weigh these strengths against their particular needs and potential drawbacks.
Oracle Autonomous Database: Features and Insights
The Oracle Autonomous Database offers significant value in the realm of cloud data warehousing. As businesses increasingly rely on data analytics for decision making, understanding the capabilities of this database solution is vital. Its autonomous nature means it can automatically manage, scale, and patch itself, reducing the need for extensive manual oversight. This aspect simplifies management for organizations, allowing IT teams to focus on strategic initiatives rather than routine operational tasks.
Automation Features
Oracle Autonomous Database leverages advanced automation to minimize human intervention. The system effectively manages routine tasks such as tuning, backups, and updates. Key aspects include:
- Self-Driving: It learns the workload and optimizes performance automatically. This means businesses can see improved efficiency without additional input from IT staff.
- Self-Securing: It includes automated security patches that protect sensitive data. This capability is increasingly important as cyber threats grow more sophisticated.
- Self-Repairing: If a failure occurs, the database can recover automatically, ensuring high availability and reliability.
These features combine to streamline database management and enhance operational efficiency for organizations.
Performance Benefits
Performance is a critical factor in selecting a database. Oracle's Autonomous Database showcases impressive capabilities in this area. By optimizing workloads and using advanced query optimization techniques, it ensures:
- High Throughput: The autonomous nature allows for faster data processing, critical for businesses with large datasets.
- Reduced Query Latency: Users often report quicker response times during data queries, which can significantly improve user experience.
- Scalability: It can scale resources up or down automatically based on workload demand, maximizing resource efficiency.
These performance benefits result in a more responsive system that evolves with organizational needs.
Cost Structure Analysis
Understanding the cost structure of Oracle Autonomous Database is essential. It typically operates on a pay-per-use model, which can be advantageous for budgeting flexibility. This model allows organizations to:
- Control Costs: Businesses only pay for the compute and storage resources they consume, avoiding fixed costs associated with traditional databases.
- Budget Predictability: As the service scales with usage, organizations can predict expenses better, allowing for better financial planning.
- Reduction in Overhead: Automation reduces the need for database administration, leading to potential savings in human resources.
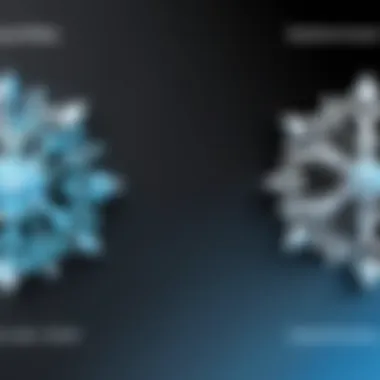
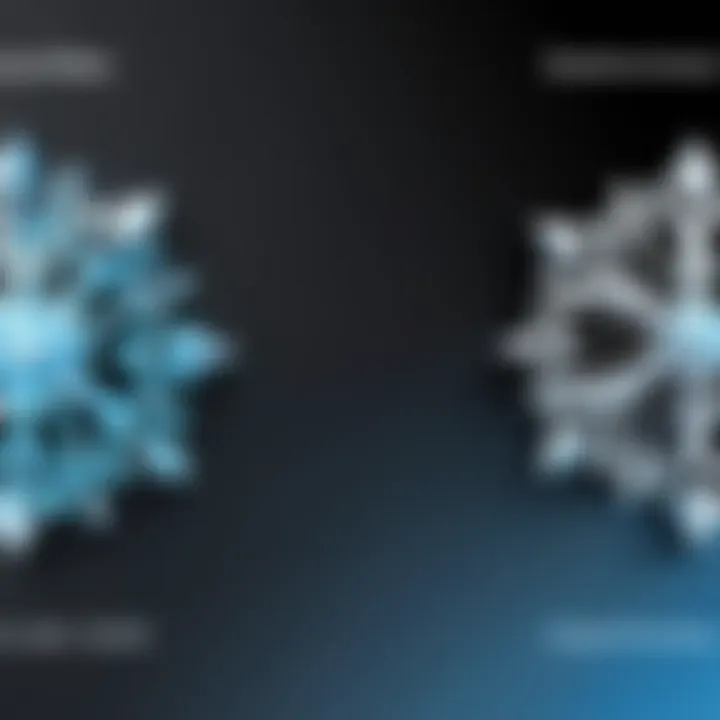
This cost structure can be particularly appealing to businesses that demand agility and efficiency.
User Experiences and Satisfaction
User feedback highlights various aspects of Oracle Autonomous Database. Many find that its automation and performance benefits translate into real-world advantages. Surveys indicate that:
- Ease of Use: Users often cite a simplified user experience due to automated tasks. This reduces the burden on IT departments.
- Satisfaction with Performance: Organizations report improved processing times and responsiveness, leading to higher satisfaction.
- Support and Community Engagement: The robust Oracle user community and available support contribute positively to overall satisfaction, as users exchange tips and troubleshoot issues effectively.
Overall, user experiences reflect a favorable view of Oracle Autonomous Database, supporting its role as a competitive offering in the cloud data warehousing market.
IBM Db2 Warehouse: Competitive Assessment
IBM Db2 Warehouse stands as a noteworthy competitor within the cloud data warehousing sector. It represents a solution rooted in IBM's formidable legacy of database management systems. Understanding its core competencies, alongside its positioning in the market, is crucial for any organization evaluating the landscape of database offerings, especially in the context of Snowflake's existing solutions. Companies aiming to harness their data effectively require clarity on what each solution can provide, and Db2 Warehouse offers unique advantages that merit attention.
Core Features and Benefits
IBM Db2 Warehouse brings several core features that cater to the diverse needs of modern businesses. One fundamental element is its ability to seamlessly integrate with other IBM products and third-party tools, fostering a smooth operational ecosystem.
- Hybrid Deployment: It supports deployment in both on-premises and cloud environments, allowing companies to maintain control over their data while also leveraging the flexibility of cloud resources.
- AI Capabilities: It incorporates AI-driven analytics capabilities, enabling businesses to derive insights from their data faster. The system can automatically optimize workloads and improve performance, thus reducing resource consumption.
Moreover, the platform offers robust security features, ensuring data privacy and compliance. Through features like data masking and encryption, organizations can maintain the integrity of sensitive information.
Performance Analysis
In terms of performance, IBM Db2 Warehouse is designed to deliver high efficiency and responsiveness. Its architecture is optimized for complex analytics, benefiting from in-memory processing and columnar storage. These characteristics allow for quicker query responses, even when handling large datasets.
Performance metrics indicate that:
- Query Performance: Users typically experience significant reductions in query response times compared to traditional databases.
- Concurrent Users: The platform can handle a high number of concurrent users without sacrificing performance, which is particularly important for enterprises with numerous departments accessing data simultaneously.
Financial Considerations
From a financial perspective, adopting IBM Db2 Warehouse requires careful evaluation of its pricing model. The subscription-based pricing structure can offer flexibility and scalability, allowing organizations to align costs with their growth trajectory. However, potential users should consider the following:
- Cost of Ownership: Organizations must assess not only the licensing fees but also additional costs related to infrastructure and maintenance.
- Long-term Commitment: Evaluating multi-year contracts may be beneficial for cost savings but requires commitment to the platform's ecosystem.
In summary, initial assessments suggest that while IBM Db2 Warehouse may require a substantial investment upfront, its potential return on investment through enhanced analytics capabilities could well justify the expenditure.
Insights from User Feedback
User feedback is invaluable in illuminating the real-world applications of IBM Db2 Warehouse. Reviews often highlight:
- Ease of Use: Many users report a steep learning curve initially due to the complexity of features. However, once acclimatized, they appreciate the intuitive interface and powerful customization options.
- Support Services: Users have mixed perceptions of IBM's customer support. Some highlight prompt assistance, while others express the need for improvements in response times.
Additionally, case studies frequently emphasize the platform's ROI in sectors like finance and healthcare, where data insights are paramount. Businesses have experienced improved operational efficiencies and better customer insights after implementing IBM Db2 Warehouse.
"The power of IBM Db2 Warehouse lies not just in its features, but in how effectively it can address specific business needs."
Analyzing Additional Competitors
In today’s rapidly changing digital landscape, understanding the broader array of options beyond Snowflake is critical for organizations looking to optimize their data strategies. Analyzing these additional competitors provides insight into diverse solutions that could better fit specific business needs. This section highlights not only established players in the market but also emerging technologies and open-source alternatives. By exploring these options, decision-makers can identify solutions that offer unique features, better pricing structures, or superior scalability. Each database mentioned here can serve different scenarios, aligning with the distinct demands of organizations.
Teradata Vantage Overview
Teradata Vantage is a well-known player in the data warehousing space, recognized for its ability to manage large volumes of data efficiently. It distinguishes itself with robust analytical capabilities and supports various data workloads. Vantage combines both traditional data warehousing features and advanced analytics in a single platform, which is appealing to many businesses.
Some notable attributes of Teradata Vantage include:
- Multi-cloud deployment: Teradata supports hybrid and multi-cloud environments, allowing organizations flexibility in choosing where to store and process their data.
- Integrated AI capabilities: The platform offers built-in tools that facilitate advanced analytics and machine learning.
- Support for diverse data types: Teradata can handle structured and unstructured data, making it versatile for various data scenarios.
Many businesses utilize Teradata Vantage for its speed and scalability, suitable for enterprises that require extensive data processing capabilities.
Snowflake Alternatives in Open Source
Open-source database options are increasingly gaining popularity as viable alternatives to proprietary systems like Snowflake. These solutions often come with lower costs and provide greater customization flexibility. They can appeal to organizations with specific needs or those that prefer to avoid vendor lock-in. Some prominent open-source alternatives include Postgres and Apache Hive.
These systems can deliver significant benefits, such as:
- Cost efficiency: Without the licensing fees of commercial products, organizations can allocate budgets more efficiently.
- Community support: Many open-source platforms have strong communities that continually improve the software and provide support.
- Customization: Organizations can modify the source code to fit their unique requirements, enabling tailored solutions.
The adoption of open-source alternatives is worth considering, especially for organizations prioritizing cost-efficiency and adaptability.
Emerging Players in the Market
The cloud data warehousing market is not stagnant; it is evolving with new entrants consistently emerging. These players often serve niche markets or offer innovative features that challenge conventional solutions. Noteworthy contenders include Starburst Data and Yellowbrick Data. Both solutions focus on addressing specific data-related challenges that organizations face today.
Key aspects that these emerging players bring to the table:
- Specialized functionality: Some of these platforms provide unique capabilities designed to handle specific workloads or types of analysis more proficiently.
- Agility: Newer platforms often adapt quickly to changes in technology and customer requirements, ensuring they remain relevant in a fast-paced environment.
- Enhanced user experience: Many newcomers emphasize user-friendly designs and interfaces, making complex processes accessible to non-technical users.
By exploring these emerging players, organizations can uncover innovative solutions that align with modern business requirements and expectations.
Comparative Summary of Features
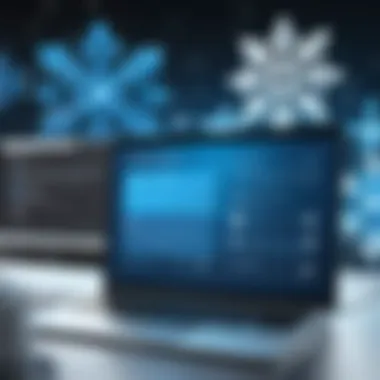
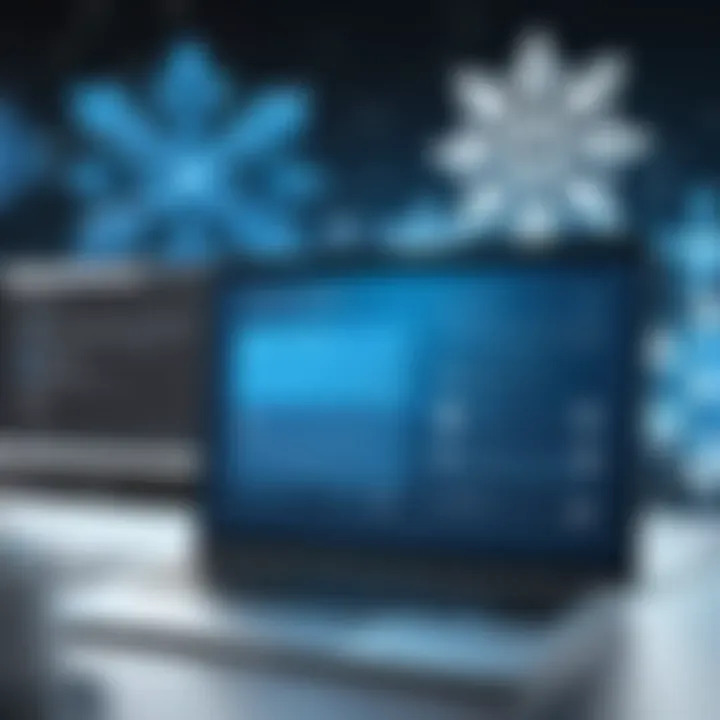
The comparative summary of features is an essential component of any analysis of Snowflake database competitors. This section distills critical information regarding each competitor’s unique offerings. A clear understanding of features can influence the decision-making process for business leaders and IT professionals.
Knowing the various features allows organizations to align their specific needs with the right technological solutions. Companies can choose more suitably based on what precise functionalities they require. Understanding features not only helps gauge a product’s capabilities but also its limitations.
Here are some key considerations during the feature comparison:
- User Interface: A well-designed interface can significantly impact user experience.
- Data Integration: The ease of integrating data from multiple sources is vital for data-driven decision-making.
- Security Features: Robust security measures are non-negotiable in today’s data management landscape.
- Analytical Capabilities: Competitive databases should provide advanced analytics tools to derive insights.
Thus, an in-depth feature set comparison facilitates a better understanding of how each competitor can serve an organization’s goals.
Feature Set Comparison
The feature set comparison gives a detailed look at the functionalities offered by various databases. Each competitor presents its own strengths and weaknesses through their specialized tools and capabilities. For instance:
- Snowflake: Allows for seamless scaling and multi-cloud solutions, ideal for diverse data workloads.
- Amazon Redshift: Focuses on reliable performance metrics, especially for businesses invested in the AWS ecosystem.
- Google BigQuery: Provides exceptional speed for large-scale data analysis, appealing to firms with extensive data sets.
- Microsoft Azure Synapse Analytics: Integrates smoothly with existing Microsoft services, making it beneficial for users already in that ecosystem.
Each platform has distinctive characteristics designed for different types of users and industries. Hence, this comparative analysis becomes crucial for informed choices.
Performance Evaluation Metrics
Performance evaluation metrics are critical to understanding how well a database functions under various conditions. These metrics can cover a range of performance aspects, including:
- Query Speed: Fast response times are essential to ensure user satisfaction.
- Concurrency: Ability to handle multiple requests simultaneously without slowdowns.
- Data Processing Capacity: Assessing how much data can be processed efficiently in a given timeframe.
For example, a user may find that Google BigQuery excels in processing large queries rapidly, whereas Amazon Redshift might serve better in scenarios with high concurrency. Evaluating these metrics enables decision-makers to select a competitor that best aligns with performance expectations.
Pricing Structures Overview
Understanding the pricing structures of competing databases is crucial when evaluating options. Different platforms may follow various pricing models, and these can impact the overall cost of ownership. Here are primary pricing considerations:
- Subscription Models: Some databases offer monthly subscriptions that include various features, providing predictable costs.
- Pay-as-You-Go Pricing: Allows users to pay based on their usage, which can help manage costs in fluctuating business environments.
- Licensing Costs: Some databases may have significant upfront licensing fees that businesses need to be aware of.
For example, Oracle Autonomous Database often utilizes a licensing model that may result in higher initial costs, but it compensates with advanced features and performance. Conversely, Google BigQuery's pay-as-you-go model may appeal to businesses with unpredictable workloads.
Evaluating pricing structures gives a clearer picture of potential financial commitments and assists organizations in budgeting effectively.
Decision-Making Considerations for Choosing a Database
In the realm of cloud data warehousing, decision-making is a critical component. Selecting the right database solution requires a deep understanding of organizational requirements, strategic goals, and the technological landscape. This section will cover essential elements relevant for making informed choices about database solutions.
Identifying Business Needs
Before evaluating different database options, organizations must first clearly identify their business needs. This process involves understanding the types of data that will be stored and processed, the volume of data, and the frequency of access.
Key considerations include:
- Data Types: Different databases specialize in various types of data management, such as structured, semi-structured, or unstructured data. It is important to choose a database that aligns with your data types.
- User Requirements: Knowing who will access the data and how often can inform decisions. For instance, if real-time analytics is essential, certain databases may perform better than others.
- Future Needs: Organizations must also consider anticipated growth. Will the data usage increase significantly in the coming years? Understanding potential future needs can help in selecting a more scalable solution.
Importance of Scalability
Scalability is another critical factor when choosing a database. As businesses grow, their data requirements evolve. It is important that the selected database can accommodate fluctuations in workload without significant redesign or major resource investments.
There are two types of scalability to consider:
- Vertical Scalability: This involves increasing the resources of existing machines. Adding CPUs or memory can be a straightforward way to increase capacity, but it does have limits.
- Horizontal Scalability: This enables distributing workloads across multiple machines. If a database supports horizontal scaling effectively, it can grow beyond the limitations of any single hardware resource.
Assessing how well a database can scale helps prevent bottlenecks that can arise from growing data and user demands. A lack of scalability can lead to degraded performance and user dissatisfaction, so it must be a priority in the decision-making process.
Understanding Total Cost of Ownership
Total Cost of Ownership (TCO) encompasses not only the initial purchase price but also the long-term costs associated with maintaining and operating the database. This includes factors such as licensing fees, hardware requirements, and ongoing support costs.
Key aspects to evaluate include:
- Licensing Models: Different databases have varied licensing structures, from pay-as-you-go to subscription fees. Analyzing these models against expected usage patterns is vital.
- Hidden Costs: Beyond licensing, consider costs that may arise from data migration, training personnel, or integrating with existing systems. It is essential to account for these hidden costs to obtain a realistic understanding of TCO.
- Operational Costs: Regular maintenance, updates, and support can accumulate over time. Understanding these recurring costs allows organizations to budget accordingly.
Ultimately, having a clear picture of the total cost of ownership will lead to better financial planning and potentially significant savings.
Culmination
The conclusion of this article on Snowflake database competitors serves as a crucial capstone. This section underscores the significance of the insights presented throughout the discussion. By wrapping up the key findings, readers can consolidate their understanding of how alternatives to Snowflake—like Amazon Redshift and Google BigQuery—align with their needs.
A well-rounded conclusion offers clarity. It emphasizes how competitive analysis facilitates better decision-making for organizations when selecting a data management solution. The importance here is not just in recognizing various competitors but understanding how their features, performance, and pricing come into play in real-world scenarios. Summarizing the pros and cons of each competitor reinforces the idea that a single solution does not fit all business models.
Ultimately, the conclusion highlights that a well-informed decision can directly affect data strategy and operational efficiency. Reliable data warehousing is not merely about technology adoption but about ensuring that the chosen system can adapt and grow with one’s organization.
Summarizing Key Findings
In our exploration, we have identified several crucial points:
- Versatile Solutions: Each competitor has its own strengths. Amazon Redshift offers excellent integration within the AWS ecosystem, while Google BigQuery shines in its serverless architecture, which enhances scalability.
- Price Variability: Pricing remains a key consideration. Understanding the total cost of ownership across these platforms is essential. For companies with varying data workloads, flexible pricing models are paramount.
- User Experiences: Feedback from current users has revealed varied levels of satisfaction, often connected to specific use cases and industry requirements.
These insights equip organizations with crucial information to make an informed decision that not only meets their immediate requirements but also positions them strategically for future growth.
Future Trends in Cloud Data Warehousing
Looking ahead, cloud data warehousing will continue to evolve. Several trends are emerging in this space that merit attention:
- Increased Automation: Many platforms are integrating more automation in data management tasks, which streamlines workflows and reduces human error.
- AI and Machine Learning Integration: Technologies like artificial intelligence and machine learning are beginning to enhance data analysis capabilities, allowing businesses to extract valuable insights more efficiently.
- Hybrid Solutions: With the rise of hybrid cloud environments, we can expect more databases to support multi-cloud strategies. This will provide businesses greater flexibility and reduced vendor lock-in.
As these trends materialize, organizations must stay informed to strategically align with their data needs. Exploring these advancements will be beneficial for optimizing data performance and capitalizing on the opportunities that arise as the market shifts.