Survey Analytics: Unveiling Data-Driven Insights
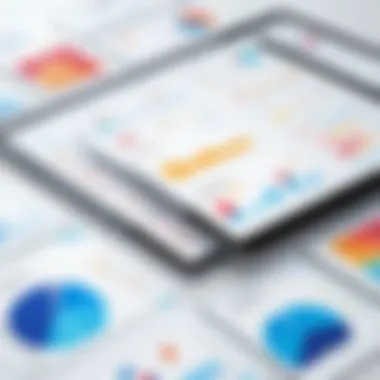
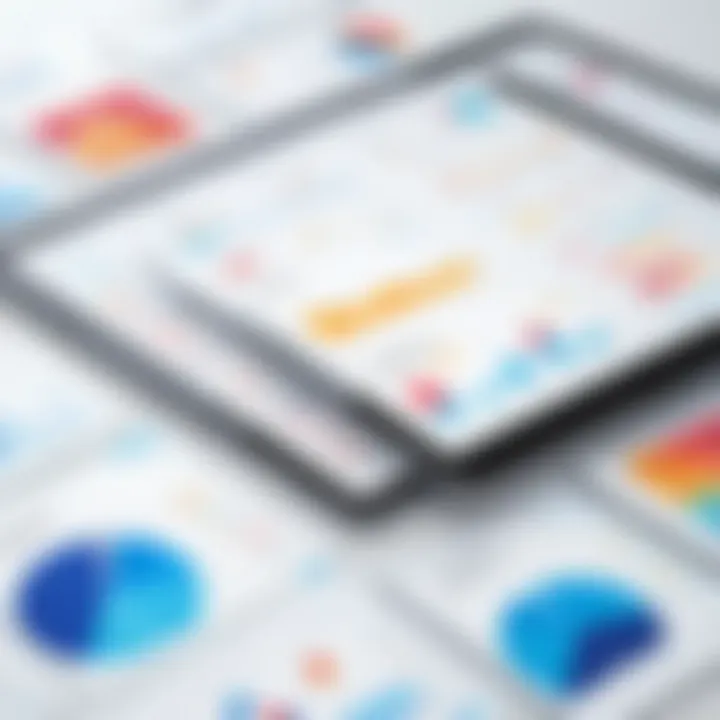
Intro
In today's fast-paced, data-driven landscape, understanding survey analytics is crucial. The ability to collect, analyze, and interpret survey data leads to informed decision-making that can significantly impact organizational success. This section provides a clear insight into the significance of survey analytics, its methodologies, tools, and the challenges professionals face in this domain.
With the rise of big data, organizations can harness survey research to obtain valuable feedback. The information derived not only helps in improving products or services but also enhances customer satisfaction and loyalty. As the methods of gathering data evolve, so do the techniques for analyzing this data. An effective approach to survey analytics is multi-faceted, integrating various tools and techniques to generate comprehensive insights.
Importance of Survey Analytics
- It aids organizations in understanding customer preferences.
- Helps in gauging employee satisfaction and engagement levels.
- Drives strategic planning and decision-making processes.
Survey analytics also confronts several ethical considerations. Issues such as data privacy, consent, and transparency in data usage demand careful attention. Thus, being equipped with the right knowledge and tools is vital for navigating these complexities.
As we delve deeper into the functionality and scalability of survey analytics tools, we will uncover how these systems meet varied user needs and adapt to changes in organizational structures and demands.
Prelude to Survey Analytics
Survey analytics is a form of data analysis that focuses on interpreting information gathered through surveys. It plays a crucial role in helping organizations understand consumer behavior, preferences, and trends. As we move deeper into a data-driven world, leveraging survey analytics becomes increasingly vital for strategic decision-making.
Defining Survey Analytics
Survey analytics can be defined as the systematic process of gathering, organizing, and analyzing data obtained from survey responses. This process involves various steps, including designing the survey, collecting responses, and employing analytical techniques to derive insights.
The definition also embodies methods to ensure data accuracy and reliability. By focusing on both qualitative and quantitative data, survey analytics provides a comprehensive view of the dataset. Key aspects include the selection of appropriate survey tools and the development of effective question formats.
Importance in Decision-Making
The significance of survey analytics in decision-making cannot be overstated. Organizations rely on insights gained from surveys to shape strategies, enhance operations, and improve customer interactions. Data-driven decisions tend to result in a better alignment with consumer needs and expectations.
Some benefits of utilizing survey analytics include:
- Informed Strategies: Businesses can create marketing strategies based on data, reducing the risk associated with guessing market demands.
- Customer Satisfaction: By understanding feedback from surveys, companies can adapt products and services to improve customer satisfaction.
- Identifying Trends: Regularly conducted surveys can highlight market trends, enabling organizations to stay ahead.
"Data-driven insights lead to more informed decision-making, ultimately resulting in improved business outcomes."
To summarize, understanding survey analytics provides organizations with tools to make informed decisions. This enables them to harness data effectively and gain a competitive edge in their respective industries.
Types of Surveys and Their Applications
Surveys are a vital tool for gathering insights and understanding various phenomena. Various types of surveys cater to different needs and reflect different methodologies. The classification of surveys aids researchers and decision-makers in selecting the most suitable approach to collect data relevant to their specific objectives. Each survey type has distinct applications and benefits that can greatly influence the outcomes of analyses.
Online Surveys
Online surveys have gained immense popularity due to their convenience and cost-effectiveness. They allow respondents to complete questionnaires at their own pace, which can lead to higher quality responses. Tools like SurveyMonkey and Google Forms facilitate the creation and dissemination of surveys to a wide audience.
The growth of the internet enables organizations to reach diverse populations without geographical limitations. Online surveys can be designed with various question formats, such as multiple-choice, rating scales, or open-ended questions, catering to different data collection needs. They are particularly useful for collecting quantitative data, which can be easily analyzed using various statistical software.
This method also allows for faster data collection and real-time analysis. However, it is important to consider the digital divide. Not everyone has equal access to the internet, potentially biasing the results.
Telephone Surveys
Telephone surveys represent another significant method of data collection. These surveys typically involve an interviewer who asks questions over the phone while recording responses. They can be useful for garnering in-depth qualitative data. Respondents may feel more comfortable discussing their views via phone rather than in written form.
Calling target audiences directly can yield higher response rates compared to online surveys. This is particularly useful in certain demographics, such as older adults, who might prefer the personal touch of a phone call. Issues like respondent fatigue can arise, leading to lower quality responses if interviews are too lengthy.
Ethical considerations must also be addressed, as unsolicited calls may intrude on privacy. Nonetheless, with proper consent and approach, telephone surveys can be a powerful tool in survey analytics.
Face-to-Face Surveys
Face-to-face surveys involve direct interaction between the interviewer and participant, whether in a public place or a controlled environment. This method often results in rich qualitative data, as interviews can be adapted in real-time based on the responses given. Observations of body language and other non-verbal cues can also provide valuable insights.
These surveys can be time-consuming and expensive, requiring trained personnel to conduct the interviews effectively. However, they often result in high engagement levels and can capture more nuanced responses. The high degree of personal interaction may encourage more honest and thoughtful answers compared to other methodologies.
Ensuring a non-biased, neutral demeanor from the interviewer is crucial. Maintaining ethical standards, such as informed consent and confidentiality, is also imperative to uphold the integrity of the survey.
Data Collection Methods
In the domain of survey analytics, data collection methods are paramount. They lay the foundation for the quality of data that is generated and subsequently analyzed. Without robust data collection methods, the validity of survey insights may be compromised. Understanding these methods enables professionals to choose appropriate strategies that suit their research objectives while maximizing the reliability of the data.
Qualitative vs. Quantitative Data
Qualitative data refers to descriptive information that is often gathered through interviews or open-ended questions. This type of data provides depth and insight into respondents' thoughts, feelings, and motivations. For instance, when conducting a survey on consumer behavior, qualitative data can reveal why people prefer one product over another. However, analyzing this type of data can be challenging, as it often requires thematic analysis and coding.
On the other hand, quantitative data is numerical and can be statistically analyzed. This kind of data typically comes from structured surveys with closed-ended questions, allowing for easy comparison and aggregation. An example is measuring customer satisfaction on a scale of 1 to 10. While quantitative data provides a broader overview and is easier to analyze, it may lack the depth of qualitative insights. The choice between these types of data often depends on the specific goals of the survey.
Sampling Techniques
Sampling techniques play a critical role in survey analytics as they affect how representative the final dataset is of the larger population. Random sampling is one common method that ensures every individual has an equal chance of being selected. This can lead to a more accurate representation of the target demographic.
Non-random sampling, like convenience sampling, is another approach. This method is often easier to execute but can introduce bias if the sample is not representative. It is essential for researchers to judiciously choose the sampling method based on their objectives.
Some widely used sampling techniques include:
- Simple Random Sampling: Every member of the population has an equal chance of being included.
- Stratified Sampling: The population is divided into subgroups or strata, and random samples are taken from each.
- Systematic Sampling: Researchers select every nth individual from a list.
Choosing the right sampling technique is crucial for ensuring the credibility and generalizability of survey results.
"The method of data collection significantly influences the insights that can be derived from survey analytics."
In summary, data collection methods are foundational in survey analytics. Understanding the nuances between qualitative and quantitative data, along with different sampling techniques, undoubtedly enhances the quality of insights obtained from survey analytics.
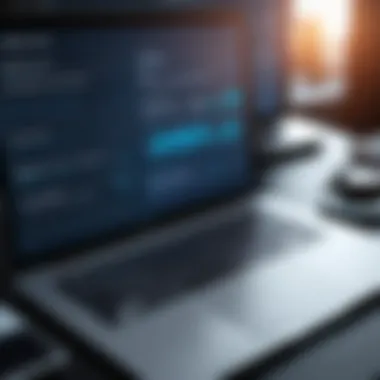
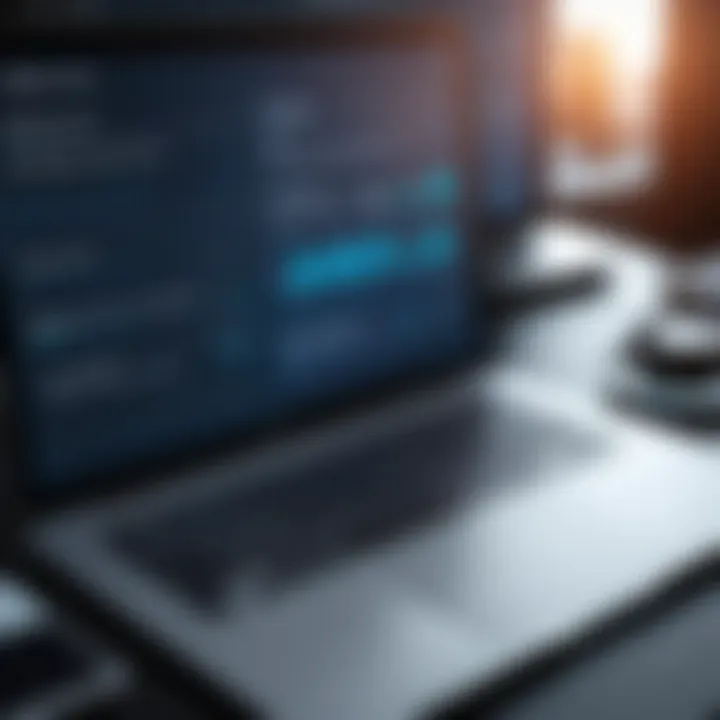
Key Components of Survey Analytics
Understanding the key components of survey analytics is crucial for effective data interpretation and actualization of insights. These components shape the overall effectiveness of a survey. They focus on the development of insights from the respondent’s feedback. When designed well, surveys can significantly influence strategic choices and operational adjustments in organizations.
Questionnaire Design
Questionnaire design is foundational in capturing relevant data. A well-structured questionnaire ensures that the questions asked are clear, concise, and capable of eliciting the necessary information. Poor design can lead to ambiguous responses, ultimately skewing the data.
Key elements of effective questionnaire design include:
- Clarity: Questions must be easy to understand. Avoid jargon or overly complex phrasing.
- Relevance: Each question should relate directly to the research objectives. Only pertinent questions should be included.
- Question Types: Use a mix of closed-ended and open-ended questions. Closed-ended questions facilitate quicker analysis, whereas open-ended questions provide richer insights.
- Logic Flow: The arrangement of questions should follow a logical order to maintain respondent engagement. Grouping similar topics can also help with coherence.
Incorporating these design aspects can deliver reliable and rich datasets for analysis. This aspect cannot be overlooked as it directly impacts the quality of gathered insights.
Response Scaling Techniques
Response scaling techniques play a critical role in how respondent feedback is measured. Selecting the appropriate scale type impacts data interpretation significantly. Common scaling techniques include:
- Likert Scale: This is a popular approach in which respondents indicate their level of agreement or satisfaction on a symmetric agree-disagree scale.
- Semantic Differential Scale: This allows respondents to rate a product or service between two bipolar adjectives. This is useful for measuring attitudes or feelings.
- Numeric Rating Scale: This method uses numbers to scale responses, often from 1 to 10, indicating overall satisfaction.
Each technique has its merits and is suitable for different types of questions. The selected scaling method should align with the purpose of the survey and the nature of the data being collected.
Selecting appropriate scaling not only enhances the precision of collected data but also ensures that the analysis reflects genuine respondent sentiments. Properly executed, response scaling can greatly contribute to the robustness of survey findings.
Analytics Tools and Software
In the realm of survey analytics, the right tools can significantly enhance the quality, efficiency, and depth of data analysis. These tools streamline the process of collecting, analyzing, and interpreting data gathered from surveys. They cater to various needs—from designing surveys to visualizing the results—making them essential for both novice and experienced data analysts. By utilizing these tools, organizations can glean actionable insights that drive decision-making processes.
Survey Management Platforms
Survey management platforms are critical for facilitating the entire survey process. They allow users to create, distribute, and manage surveys efficiently. Platforms like SurveyMonkey and Qualtrics offer user-friendly interfaces and robust features. These features typically include customizable templates, question logic, and respondent tracking.
One of the key benefits of using such platforms is the ability to collect data in real-time. This ensures a quicker turnaround for analysis. Additionally, many of these platforms integrate seamlessly with analytical tools, allowing for a smooth transfer of data. Overall, they save time and resources, enabling businesses to focus on deriving insights rather than managing logistics.
Statistical Analysis Software
Statistical analysis software is essential for in-depth data analysis. Programs like SPSS and R offer sophisticated statistical techniques that enable researchers to perform complex data analyses. These software solutions provide various functionalities, such as hypothesis testing, regression analysis, and advanced statistical modeling.
Moreover, using proper statistical analysis helps ensure the reliability of findings. Poor analysis can lead to misleading conclusions, potentially skewing business strategies. By employing robust software, analysts can uncover nuanced trends and relationships within the data. This leads to a more informed understanding of customer preferences and market behaviors.
Data Visualization Tools
Data visualization tools play an indispensable role in the analysis of survey data. Tools such as Tableau and Microsoft Power BI enable users to present data in a visually engaging manner. They transform raw data into clear, understandable charts and graphs, facilitating better decision-making.
Visual representations of data not only improve comprehension but also enhance the ability to communicate findings to stakeholders. For instance, stakeholders may easily spot trends, outliers, and correlations presented graphically rather than in complex tables. Effective data visualization helps ensure that survey insights are accessible and actionable.
"The right visualization can make complex data comprehensible, turning mere numbers into valuable insights."
Analyzing Survey Data
Analyzing survey data is a crucial step in transforming raw numbers into actionable insights. This process allows researchers and decision-makers to understand patterns and trends in respondents' feedback. By carefully analyzing survey data, one can derive significant conclusions that support business strategies and policy formulations.
Descriptive Statistics
Descriptive statistics provide a summary of the data collected through surveys. Techniques in this category include measures like mean, median, mode, and standard deviation. These statistics help in simplifying large datasets, making them easier for stakeholders to understand. For example, a mean score for customer satisfaction can instantly reveal whether a product meets quality expectations.
Furthermore, descriptive statistics can be visually represented using graphs and charts, which enhances comprehension. Here are some common visual tools:
- Bar Charts
- Pie Charts
- Histograms
- Box Plots
By utilizing these tools, organizations can communicate data findings more effectively. This step is vital for identifying trends and making data-driven decisions.
Inferential Statistics
Inferential statistics go a step further by allowing researchers to make predictions or inferences about a larger population based on sample data. This branch of statistics uses techniques like hypothesis testing, confidence intervals, and regression analysis. For instance, if a survey collected responses from 500 users, inferential statistics could enable a company to generalize findings to a target market of thousands.
The significance of inferential statistics is particularly evident when it comes to decision-making. By understanding potential variances, organizations can better allocate resources and refine strategies.
"Inferential statistics help translate sample data into insights about broader trends, enabling businesses to make informed decisions."
Advanced Analytical Techniques
Advanced analytical techniques provide deeper insights that go beyond basic statistics. Techniques such as multivariate analysis, cluster analysis, and text analytics can unveil hidden relationships in the data. For instance:
- Multivariate Analysis: This method examines the relationship between multiple variables simultaneously. It can provide insights into factors affecting customer behavior.
- Cluster Analysis: This technique groups similar respondents together, facilitating the identification of specific market segments.
- Text Analytics: This approach analyzes open-ended responses to extract themes or sentiment, which is valuable in understanding customer opinions in depth.
These advanced techniques are particularly important in complex datasets where simple averages may not capture the nuances involved. Employing these methods can critically enhance the effectiveness of survey analytics and yield richer data interpretations.
Visualizing Survey Results
The visualization of survey results is a crucial component of survey analytics. It allows for the transformation of raw data into comprehensible and actionable information. Effective data visualization can uncover hidden patterns and trends. This makes it easier for decision-makers to grasp insights quickly, facilitating a more informed decision-making process.
Importance of Data Presentation
Presenting data in a clear format enhances communication of findings. It allows stakeholders to engage with the data at a deeper level. Poor presentation can lead to misinterpretation or complete disregard for valuable insights. Key aspects include:
- Clarity: Well-structured visuals provide immediate understanding.
- Engagement: Engaging visuals capture attention and motivate action.
- Comparison: Visual formats allow for easy comparison between different data sets.
Graphs, charts, and infographics serve to summarize complex survey results simply. A study indicated that people recall information better when it is presented visually rather than in text. Thus, proper data presentation improves retention and application of insights gained from surveys.
"Good data visualization helps to tell a story and present findings that can lead to meaningful actions."
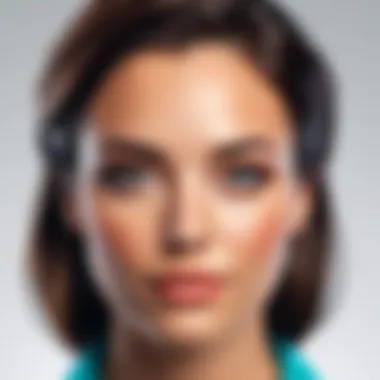
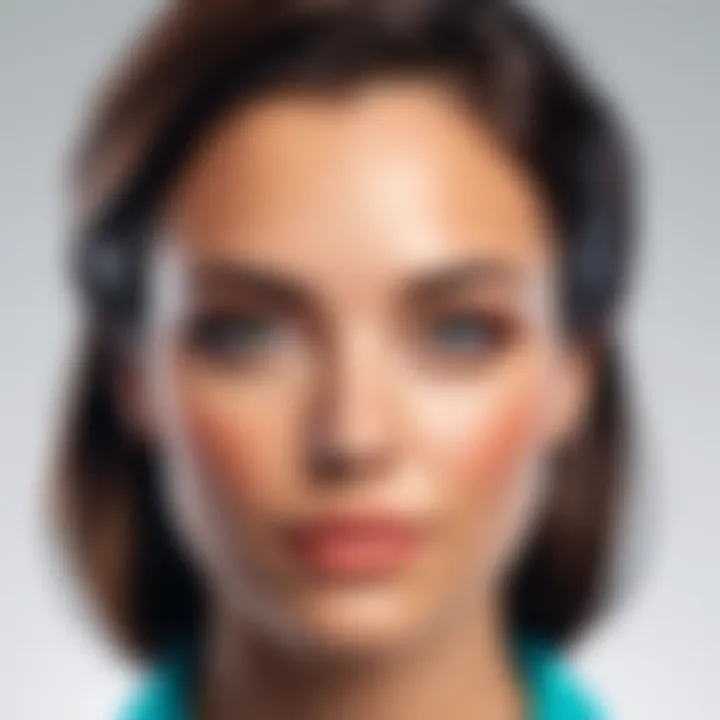
Common Visualization Techniques
Several visualization techniques are commonly used to present survey results effectively. Each method carries its unique strengths suited for specific types of data. Key techniques include:
- Bar Charts: Ideal for comparing quantities across discrete categories.
- Pie Charts: Useful for displaying proportional data, offering a quick view of how parts contribute to a whole.
- Line Graphs: Best for showing trends over time, allowing for continuous data analysis.
- Heat Maps: Provide insight into data density and relationships among variables using color gradients.
- Dashboards: Combine multiple visualizations in one interface for an integrated overview of survey findings.
Utilizing these techniques helps convey insights in a meaningful way. Selecting the right visualization is essential for enhancing comprehension and ensuring that the data tells an effective story.
Case Studies in Survey Analytics
Case studies in survey analytics play a pivotal role in understanding the practical implementations and outcomes of survey methods in various sectors. They provide real-world examples that highlight not only the effectiveness of different analytics strategies but also the challenges faced by organizations. Such case studies offer valuable insights into how data-driven decisions can lead organizations towards their goals. By examining specific applications of survey analytics within both the business and non-profit sectors, we can derive lessons that are applicable across diverse fields.
Business Sector Applications
In the business landscape, survey analytics offers a myriad of applications that can significantly influence decision-making. Companies leverage surveys to gauge customer satisfaction, employee engagement, and market trends. For instance, a multinational retail corporation may implement a customer feedback survey after a recent product launch. Analyzing this survey data helps them identify customer preferences and potential areas for improvement. This type of data-driven approach enables businesses to adapt their strategies based on actual consumer feedback rather than assumptions.
Another notable example is a financial services firm employing survey analytics to assess client satisfaction regarding their services. By analyzing the data collected from these surveys, they can pinpoint specific issues that may deter client retention. Additionally, these insights assist the firm in tailoring services that align more closely with customer needs, subsequently leading to improved satisfaction and loyalty.
Non-Profit Sector Applications
The non-profit sector benefits greatly from survey analytics as well. Organizations often rely on surveys to understand community needs, measure the impact of their programs, and gather feedback from stakeholders. For example, a health-focused non-profit may distribute surveys to assess the efficacy of its health awareness campaigns in a specific community. Analyzing the feedback allows them to modify their approach to better serve the community's needs.
Moreover, surveys can help non-profits engage their donors and volunteers effectively. By conducting surveys that capture volunteer experiences and donor motivations, these organizations gain insight into what drives engagement. This data can refine outreach strategies and enhance relationships with key stakeholders, ultimately leading to more successful campaigns and fundraising efforts.
"Understanding how survey data translates into actionable strategies is essential for both profit and non-profit sectors."
In summary, case studies in survey analytics reveal the critical role that surveys play in strategizing and optimizing operations across sectors. Through direct applications, organizations can not only enhance their performance but also ensure they remain responsive to the needs of their clients and communities.
Challenges in Survey Analytics
In the field of survey analytics, understanding the challenges is essential. Data quality and respondent bias represent two significant hurdles that can impact the validity of survey results. Addressing these issues allows for improvements in insights derived from survey data, ultimately enhancing decision-making and strategic initiatives. An organization that fully comprehends these challenges is better positioned to implement effective collection strategies and build robust data interpretation frameworks.
Data Quality Issues
Data quality encompasses various factors, such as accuracy, reliability, and relevance of the data collected. If data quality is poor, it can lead to misguided decisions based on flawed information. Common issues related to data quality include:
- Incomplete Responses: Some respondents may skip questions or fail to provide sufficient detail, leading to gaps in data analysis.
- Inconsistencies: Responses may be contradictory, suggesting misunderstanding of questions or lack of attention. This can distort analytics and paint a misleading picture of trends and preferences.
- Data Entry Errors: Human mistakes can occur during data input, which can alter results significantly.
To combat these issues, organizations should employ validation techniques during data collection, like filtering for outlier responses and ensuring that each entry is consistent with the expected format and context. Implementing training for survey administrators may also enhance data collection quality, ensuring respondents fully grasp the purpose and structure of the survey.
Respondent Bias
Respondent bias occurs when individuals' answers are influenced by various factors, leading to a misrepresentation of true feelings or opinions. Such biases complicate the interpretation of survey results and can skew data analysis. Various types of respondent bias include:
- Social Desirability Bias: Respondents may present themselves in a favorable light, answering questions based on what they believe is socially acceptable rather than their true thoughts.
- Recency Bias: This arises when respondents overemphasize their most recent experiences, neglecting earlier relevant experiences which better assess the situation.
- Confirmation Bias: Individuals may tend to focus more on questions that reinforce their existing beliefs, thus filtering their responses through a biased lens.
To mitigate respondent bias, researchers can design surveys with neutral wording and ensure that questions are balanced. Offering anonymity can also help participants feel safer in displaying their honest opinions, leading to more credible data collection.
"Cause and effect analyses in survey analytics can only be accurate when data quality and respondent bias are actively managed."
Ethical Considerations in Survey Analytics
Understanding the ethical implications in survey analytics is crucial. As organizations increasingly rely on data-driven strategies, they must also navigate the complex landscape of ethics. The integrity of survey analytics not only ensures compliance with laws but also builds trust with participants. Key elements include informed consent and protection of data privacy. By prioritizing ethical considerations, businesses can enhance their reputations and improve the quality of the data collected.
Informed Consent
Informed consent is a foundational principle in survey research. It mandates that participants understand what they are participating in. They need to know the purpose of the survey, how the data will be used, and their rights. Ensuring informed consent involves providing clear and accessible information prior to participation.
Benefits of proper informed consent include:
- Increased response rates due to transparency.
- Preservation of participant autonomy.
- Reduced likelihood of ethical violations or backlash.
Ensuring that all aspects of consent are clearly communicated helps in establishing a trustworthy relationship between researchers and participants. A strong informed consent process also reflects well on the organization, demonstrating a commitment to ethical practices.
Data Privacy Laws
Data privacy laws are essential for protecting individuals’ personal information. The General Data Protection Regulation (GDPR) and the California Consumer Privacy Act (CCPA) are examples of regulations that set clear guidelines on data usage and privacy. Organizations must stay compliant to avoid severe penalties.
Key aspects of data privacy laws include:
- Data minimization: Only collect data that is necessary for the research purpose.
- Data security: Implement measures to protect collected data from breaches.
- Participant rights: Allow participants to access, modify, or delete their personal information.
Complying with these laws not only serves to protect consumers but also bolsters the organization’s credibility. Adhering to ethical standards in data privacy is crucial in today’s data-centric world, where trust can often be as valuable as the data itself.
"Ethics in survey analytics shapes the future of data-driven decision-making. Without ethical considerations, the foundation of trust erodes."
In summary, ethical considerations in survey analytics are vital for ensuring responsible data handling practices. By focusing on informed consent and adhering to data privacy laws, organizations can foster trust, protect participants, and facilitate a culture of ethical data usage.
Emerging Trends in Survey Analytics
Survey analytics is an evolving field, where the integration of technology reshapes traditional practices. Understanding emerging trends is vital for leveraging data efficiently and gaining actionable insights. Continuous development in this discipline underscores its significance in the decision-making processes of modern organizations. The ability to adapt to new methodologies and tools ensures that companies remain competitive in their respective markets.
AI and Machine Learning Integration
The advent of artificial intelligence (AI) and machine learning in survey analytics is a significant trend reshaping data interpretation. These technologies enhance the predictive capabilities of analytics, enabling organizations to forecast trends based on historical data patterns.
By utilizing machine learning algorithms:
- Data Processing Efficiency: Organizations can analyze vast datasets quickly, significantly reducing the time from data collection to insight generation.
- Enhanced Data Accuracy: AI helps identify patterns and anomalies in the data that humans may overlook, improving overall data quality.
- Customized Insights: Organizations can generate tailored insights for specific audience segments, enhancing the effectiveness of surveys.
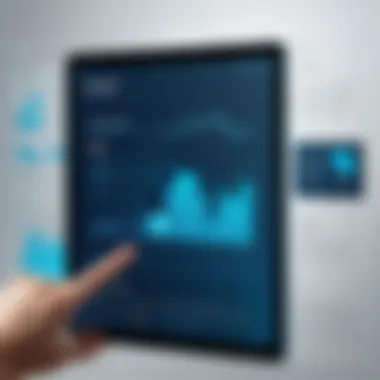
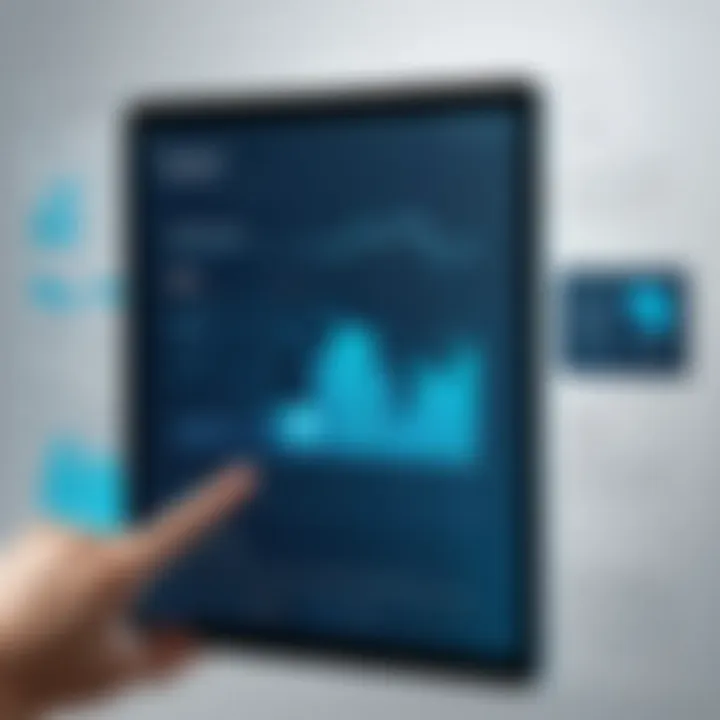
Tools such as IBM Watson and Google Cloud AI are facilitating these innovations, allowing businesses to harness advanced analytics capabilities without requiring extensive technical know-how.
Real-Time Analytics
Real-time analytics marks another critical advancement in survey analytics. The ability to analyze data as it is collected, rather than waiting for a complete dataset, offers substantial benefits. This immediacy allows organizations to make quick decisions based on current trends and immediate feedback.
The advantages of real-time analytics include:
- Timely Decision-Making: Organizations can respond swiftly to emerging trends, enhancing their agility.
- Improved Customer Engagement: Immediate responses to survey feedback foster stronger relationships with customers by showing them that their opinions are valued.
- Dynamic Adjustments: Businesses can modify marketing strategies and operational plans in real-time based on user feedback and preferences.
The rise of cloud computing and advanced data processing technologies has made real-time analytics more accessible. Platforms such as Tableau and Microsoft Power BI enable organizations to harness these capabilities seamlessly.
"In the world of survey analytics, staying updated with emerging trends is not just beneficial; it is essential for survival and growth."
Overall, embracing these trends enhances the effectiveness of survey analytics, equipping organizations with tools necessary to navigate a complex data landscape efficiently.
Future Directions in Survey Analytics
As the landscape of data analytics evolves, the future directions in survey analytics gain significant importance. Organizations must stay attuned to emerging trends to capitalize on the rich insights that survey data can provide. New methodologies and technologies are reshaping how surveys are designed, conducted, and analyzed. This section illuminates key elements that will steer the future development of survey analytics.
Personalization of Surveys
Personalization stands out as a pivotal trend in survey analytics. Tailoring surveys to fit the unique preferences and behaviors of respondents leads to higher engagement rates and richer data quality. By leveraging demographic information, historical data, and respondent behavior, companies can create customized survey experiences. This approach ensures that questions are relevant to the target audience, increasing the likelihood of accurate and insightful responses.
Benefits of personalized surveys include:
- Higher Response Rates: Customized questions resonate better with respondents, encouraging participation.
- Improved Data Quality: By addressing individual needs, the data collected becomes more reflective of the true opinions and behaviors of the audience.
- Enhanced Customer Experience: Providing a personalized survey experience can enhance brand loyalty and customer satisfaction.
Cross-Platform Analysis
In a world where users interact across various devices and platforms, cross-platform analysis emerges as a crucial direction for survey analytics. It involves collecting and analyzing data from different sources, ensuring a holistic view of the respondent.
The importance of cross-platform analysis can be summarized through several facets:
- Unified Insights: Aggregating data from multiple platforms gives a comprehensive understanding of trends and consumer behavior.
- Behavioral Tracking: Tracking how respondents interact with surveys across different channels helps in optimizing future strategies.
- Data Integration: Combining insights from various sources facilitates deeper analysis and supports more nuanced decision-making.
The ability to adapt to various devices will continue to shape survey methodologies, ensuring that organizations effectively reach their audience where they are most engaged.
"The evolution towards personalization and cross-platform analytics represents a significant leap in our ability to understand consumer behavior and preferences."
By understanding these future directions, businesses can harness survey analytics’ full potential, fostering improved decision-making and strategic initiatives.
Practical Applications of Survey Analytics
Survey analytics plays a vital role in various sectors, allowing organizations to gather meaningful data that drives decisions and strategies. This section explores the practical applications of survey analytics in real-world scenarios, clarifying its importance in understanding customer needs, enhancing products, and shaping the future direction of businesses.
Enhancing Customer Experience
Understanding customer experience is crucial for businesses looking to thrive in today's competitive landscape. Survey analytics helps organizations capture feedback directly from users. This input can highlight areas needing improvement, which is essential for tailoring services or products to meet customer expectations.
Surveys can be distributed at various customer touchpoints. For instance:
- Post-purchase surveys assess satisfaction and identify potential issues.
- Experience surveys gauge users’ interaction with digital platforms.
- Continuous feedback loops maintain a pulse on customer sentiment over time.
This approach delivers actionable insights that influence several areas:
- Service improvement: Identifying pain points can help organizations enhance their offerings.
- Personalization: By analyzing customer preferences, businesses can create targeted marketing strategies.
- Loyalty building: Engaging with customers and showing that their opinions matter can foster long-lasting relationships.
The use of tools like Zoomerang or Typeform allows for the seamless collection of feedback. Furthermore, employing data visualization techniques can clarify survey results, making it easier for decision-makers to understand customer trends.
"Informed decisions lead to improved experiences and sustained customer loyalty."
Informing Product Development
Product development is another critical area where survey analytics aids organizations. By incorporating customer feedback, businesses can adapt their product offerings to better match market demands. Surveys serve as a channel to gauge interests and desires effectively.
Conducting surveys during different product development phases provides valuable insights:
- Concept testing: Early feedback on ideas can validate potential product features.
- Prototype testing: Gauging user reactions to prototypes helps refine designs before launch.
- Post-launch evaluations: Understanding customer acceptance and satisfaction helps identify areas for future improvements.
Several key benefits emerge from this process:
- Informed decision-making: Utilizing data ensures that product decisions are based on empirical evidence rather than assumptions.
- Risk reduction: Early identification of potential issues can help mitigate risk associated with product failures.
- Market alignment: Surveys can help ensure that new products align with consumer expectations, ultimately leading to better sales performance.
Viewing survey data through platforms like Google Forms or Qualtrics aids in streamlining feedback collection and analysis. This leads to a more efficient product development cycle and increases the likelihood of product success in a competitive marketplace.
The Ends About Survey Analytics
The conclusions drawn from an exploration into survey analytics encapsulate the essence of effective data-driven decision-making. This article highlights that survey analytics is not merely about collecting data; it's about interpreting that data to derive actionable insights. With the rapidly evolving landscape of technology and consumer behavior, businesses must leverage survey analytics to remain relevant and competitive.
Summarizing Key Findings
Survey analytics offers myriad benefits that can transform the way organizations approach their strategies. Key findings include the following:
- Comprehensive Understanding: Organizations gain a deep understanding of customer attitudes and preferences. This information is crucial for tailoring services and products to meet those needs.
- Enhancing Engagement: Well-structured surveys promote engagement with customers. They create a two-way communication channel that enables businesses to listen and act on feedback.
- Informed Decision-making: The data collected informs critical decisions. From product development to marketing strategies, insights from surveys can significantly reduce risks associated with new initiatives.
Moreover, the importance of good questionnaire design, analyzed methodologies, and tools cannot be overstated in yielding high-quality insights. In summary, all aspects properly executed lead to results that are both quantifiable and meaningful.
Reflecting on the Role of Survey Analytics
Survey analytics plays a pivotal role in shaping business strategies and policies. It is not just a tool for feedback; it is an integral part of an organization’s strategic framework. The role of survey analytics includes:
- Data-Driven Culture: Encouraging a shift towards data-centric decision-making. Organizations that embrace survey findings are likely to foster a culture that values evidence over intuition.
- Customization and Personalization: As businesses strive for personalized experiences, survey analytics can provide tailored insights that align product offerings with individual consumer needs.
- Adaptability: Survey analytics enables businesses to adapt to market trends and shifts in consumer preferences effectively. Continuous feedback loops keep organizations agile in their responses.
"Utilizing survey analytics transforms raw data into critical insights that can guide future actions and strategies."
Conclusively, recognizing the multifaceted role of survey analytics allows businesses to harness its full potential. The insights derived foster innovation and drive sustainable growth while addressing customer needs more effectively.